Residential electricity load prediction method based on multi-model fusion, medium and equipment
A technology for residential electricity consumption and load forecasting, applied to load forecasting in AC networks, biological neural network models, electrical components, etc. , to achieve the effect of improving prediction accuracy, overcoming difficulties in accurate prediction, and ensuring safe scheduling and optimization
- Summary
- Abstract
- Description
- Claims
- Application Information
AI Technical Summary
Problems solved by technology
Method used
Image
Examples
Embodiment Construction
[0037] The present invention will be described in detail below in conjunction with the accompanying drawings and specific embodiments. This embodiment is carried out on the premise of the technical solution of the present invention, and detailed implementation and specific operation process are given, but the protection scope of the present invention is not limited to the following embodiments.
[0038] Such as figure 1 As shown, the present invention provides a residential electricity load forecasting method based on multi-model fusion, which integrates multiple forecasting models to effectively improve the scope of application and forecasting accuracy. In this embodiment, the prediction models used include a gray scale prediction model, a Gaussian process regression model, and a long-short-term memory network model. to take advantage of the known residential electricity consumption x i , i=1, 2,..., t, taking the prediction of residential power consumption at time t+1 as a...
PUM
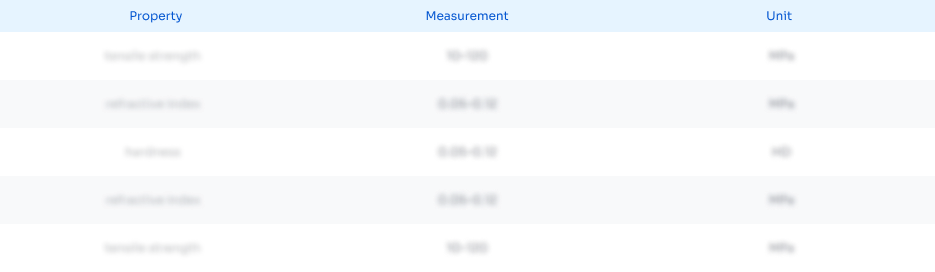
Abstract
Description
Claims
Application Information
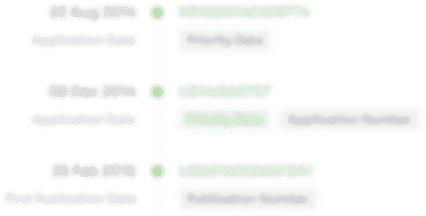
- R&D Engineer
- R&D Manager
- IP Professional
- Industry Leading Data Capabilities
- Powerful AI technology
- Patent DNA Extraction
Browse by: Latest US Patents, China's latest patents, Technical Efficacy Thesaurus, Application Domain, Technology Topic, Popular Technical Reports.
© 2024 PatSnap. All rights reserved.Legal|Privacy policy|Modern Slavery Act Transparency Statement|Sitemap|About US| Contact US: help@patsnap.com