A Semantic Segmentation Method and a Road Waterlogging Detection Method and Device Applying It
A technology for semantic segmentation and road water accumulation, applied in the field of computer vision, can solve problems such as difficulty in establishing long-distance dependencies, and achieve efficient governance, ensure stability, and the purpose and advantages are concise and easy to understand.
- Summary
- Abstract
- Description
- Claims
- Application Information
AI Technical Summary
Problems solved by technology
Method used
Image
Examples
Embodiment 1
[0047] This embodiment provides a semantic segmentation method, which inputs an image to be processed into a semantic segmentation model to obtain a corresponding semantic segmentation result.
[0048] In this embodiment, the structure of the semantic segmentation model is as follows figure 1 As shown, it includes a backbone network, a multi-scale feature extraction network and a semantic segmentation prediction head. The multi-scale feature extraction network includes a plurality of parallel global feature extraction networks, wherein each of the global feature extraction networks includes at least a series of One or more global feature extraction modules, the global feature extraction modules are CNN modules embedded with multiple stacked global attention modules;
[0049] the backbone network for extracting a first feature map of the to-be-processed image, wherein the first feature map is a feature map with local attention;
[0050] The global feature extraction network is...
Embodiment 2
[0086] This embodiment provides a road water detection method, which is implemented by applying the semantic segmentation method in Embodiment 1, and includes the following steps: acquiring an image to be processed; acquiring the image to be processed according to the semantic segmentation method described in Embodiment 1 The semantic segmentation result; identifying the road stagnant existing in the image to be processed according to the semantic segmentation result.
[0087] Before using the semantic segmentation model in this method to detect road water, the model needs to be trained.
[0088] First, collect sample images. Image collectors use mobile phones to collect long-range road water images and close-range road water images as sample images; the sample images of different types are divided into two columns and placed in the table. For the water accumulation image of the long-range road, the image collectors put the collected sample images in the table on a daily basi...
Embodiment 3
[0095] Based on the same concept, this embodiment provides a road water detection device for implementing the road water detection method described in the second embodiment, and the device includes the following units:
[0096] an acquisition unit, used to acquire the image to be processed;
[0097] a semantic segmentation unit, configured to obtain the semantic segmentation result of the to-be-processed image according to the semantic segmentation method described in Embodiment 1;
[0098] An identification unit, configured to identify road water existing in the to-be-processed image according to the semantic segmentation result.
PUM
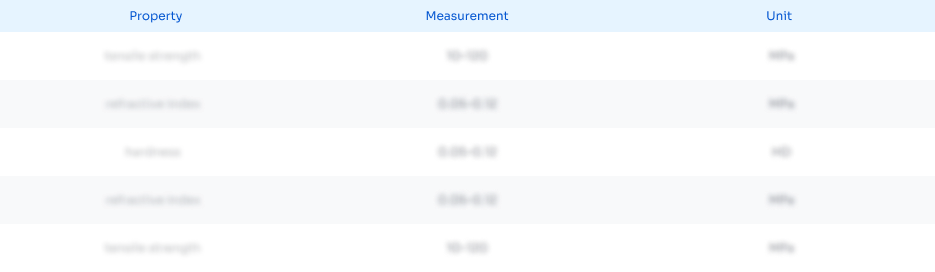
Abstract
Description
Claims
Application Information
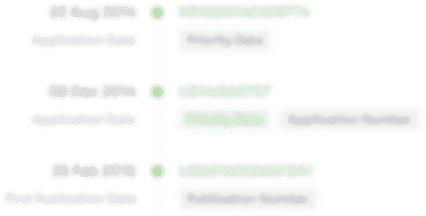
- R&D Engineer
- R&D Manager
- IP Professional
- Industry Leading Data Capabilities
- Powerful AI technology
- Patent DNA Extraction
Browse by: Latest US Patents, China's latest patents, Technical Efficacy Thesaurus, Application Domain, Technology Topic, Popular Technical Reports.
© 2024 PatSnap. All rights reserved.Legal|Privacy policy|Modern Slavery Act Transparency Statement|Sitemap|About US| Contact US: help@patsnap.com