Deep Bayesian network modeling training method
A technology of Bayesian network and training method, which is applied in the fields of data mining and artificial intelligence, and can solve problems such as poor robustness, lack of interpretability, lack of original data modeling reasoning ability, etc., and achieve good interpretability Effect
- Summary
- Abstract
- Description
- Claims
- Application Information
AI Technical Summary
Problems solved by technology
Method used
Image
Examples
Embodiment Construction
[0066] The present invention will be further described below in conjunction with accompanying drawing.
[0067] Such as figure 1 , 2 , 3, a deep Bayesian network modeling training method, using deep learning to have a strong automatic feature extraction ability, overcome its lack of interpretability, poor robustness, easy to be deceived and rely on a large number of training samples, The Bayesian network can effectively combine expert knowledge and has a complete mathematical theoretical basis, good interpretability, and can model and reason the feature quantities obtained through feature engineering. The present invention combines the advantages of both, combining deep learning with Bayesian The effective integration of the Yeesian network can obtain a "deep Bayesian network" - a Bayesian network model with automatic feature extraction capabilities, and its automatic feature extraction capabilities are obtained by relying on deep learning models, such as figure 1 shown.
...
PUM
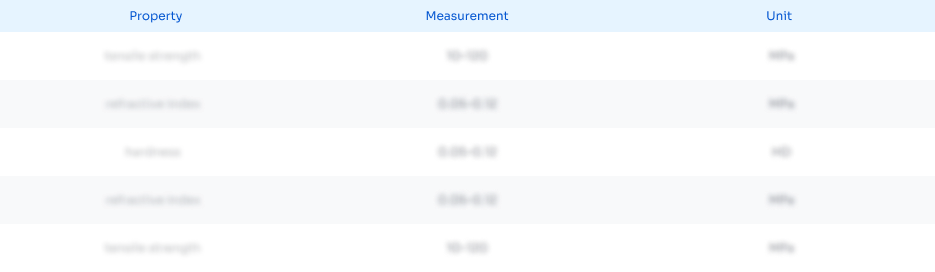
Abstract
Description
Claims
Application Information
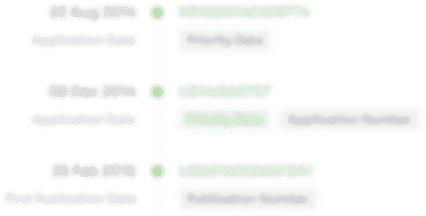
- Generate Ideas
- Intellectual Property
- Life Sciences
- Materials
- Tech Scout
- Unparalleled Data Quality
- Higher Quality Content
- 60% Fewer Hallucinations
Browse by: Latest US Patents, China's latest patents, Technical Efficacy Thesaurus, Application Domain, Technology Topic, Popular Technical Reports.
© 2025 PatSnap. All rights reserved.Legal|Privacy policy|Modern Slavery Act Transparency Statement|Sitemap|About US| Contact US: help@patsnap.com