Depth ordered regression model-oriented generative feature ordered regularization method
A technology of regression model and regression method, which is applied in the direction of neural learning method, character and pattern recognition, biological neural network model, etc., can solve the problem of damaging the essential goal of deep order regression method, and achieve the effect of improving performance
- Summary
- Abstract
- Description
- Claims
- Application Information
AI Technical Summary
Problems solved by technology
Method used
Image
Examples
experiment example 1
[0064] Experimental example 1: Quantization performance comparison in different scenarios
[0065] Table 1 Performance comparison of different methods in face age estimation
[0066]
[0067] Table 1 Performance comparison of different methods in medical image classification
[0068]
[0069] For quantitative comparisons, experimental comparisons are performed between some state-of-the-art ordinal regression methods and their combinations with our PnP-FOR. In Table 1, we mainly compare four baseline methods and their combinations with PnP-FOR: Mean-Variance [4], Poisson [9], SORD [6] and POE [5]. Notably, the proposed PnP-FOR improves over existing methods in terms of MAE, CS and accuracy. Mean-Variance and POE focus on learning the uncertainty of neural network output vectors and latent space features, respectively. Mean-Variance computes the mean loss and variance loss of the output vectors to control for uncertainty in the output space. PnP-FOR+Mean-Variance achie...
experiment example 2
[0075] Experimental example 2: Comparison of t-SNE visualization results of different methods
[0076] In order to further highlight the superiority of PnP-FOR, we have image 3 Feature representations of the four baseline methods with and without PnP-FOR are visualized by t-SNE in . It can be seen that the traditional OR method shows a certain ordered distribution in the feature space, while POE learns a relatively regular ordered distribution. Although their learning strategies can model ordered relations in latent spaces, they cannot guarantee global ordinal relations and lack intraclass compactness ( image 3 at the red arrow).
[0077] Using our PnP-FOR, all results can be tuned to a nearly one-dimensional space, which is consistent with the real ordered label space, which also verifies the effectiveness of our motivation. Moreover, PnP-FOR not only preserves the local order relation, but also preserves the global distribution. On the other hand, PnP-FOR encourages in...
experiment example 3
[0078] Experimental Example 3: Effects of Different Sampling Strategies on PnP-FOR
[0079] We investigate two sampling strategies for batch training using PnP-FOR: 1) random sampling (Random), and 2) stratified sampling (Stratified), where all samples in a mini-batch have different labels. Figure 4 It shows that the results of stratified sampling are not as good as random sampling.
[0080] Stratified sampling causes SORD to slightly overfit after 25 epochs. This can be explained from two aspects. First, as shown in Equation (6), stratified sampling will pay more attention to the distance between adjacent ordered labels, because distant labels hardly affect the probability size, while random sampling contributes to the compactness between classes . Second, the probabilities in Equation (6) approximate the probabilities of batch samples. Therefore, stratified sampling is difficult to learn the true distribution of the data set.
PUM
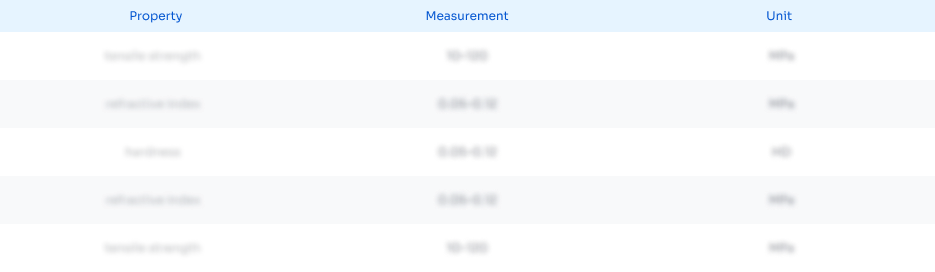
Abstract
Description
Claims
Application Information
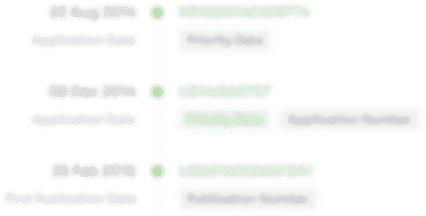
- Generate Ideas
- Intellectual Property
- Life Sciences
- Materials
- Tech Scout
- Unparalleled Data Quality
- Higher Quality Content
- 60% Fewer Hallucinations
Browse by: Latest US Patents, China's latest patents, Technical Efficacy Thesaurus, Application Domain, Technology Topic, Popular Technical Reports.
© 2025 PatSnap. All rights reserved.Legal|Privacy policy|Modern Slavery Act Transparency Statement|Sitemap|About US| Contact US: help@patsnap.com