Image style migration model training method, system and device and storage medium
A training method and image technology, applied in computing models, character and pattern recognition, machine learning, etc., can solve the problems of deformation style, no obvious improvement, content leakage, etc., and achieve the effect of improving ability and enhancing fidelity
- Summary
- Abstract
- Description
- Claims
- Application Information
AI Technical Summary
Problems solved by technology
Method used
Image
Examples
Embodiment 1
[0033] An embodiment of the present invention provides a training method for an image style transfer model, which is used to further improve the quality of image style transfer and improve the accuracy of downstream tasks. Aiming at the common problems of object structure deformation and semantic content mismatch in existing methods, the present invention adopts the mainstream encoder-decoder generator structure and the idea of adversarial learning to establish a style transfer model, and uses the cycle consistency loss constraint model training process. At the same time, a new positive and negative sample selection method is proposed, which improves the fit between contrastive learning and style transfer tasks, and makes the contrastive learning method better applied to the transfer model. The category information on which new positive and negative samples are selected is determined by the image block classification results obtained by the weakly supervised semantic segment...
Embodiment approach
[0039] This section mainly calculates three types of losses, and the preferred implementation method for calculating each type of loss is as follows:
[0040] 1) Calculate the total confrontation loss: the first generator uses the input source domain image to generate the target domain image, and the first discriminator is used to judge whether the input image is the target domain image generated by the first generator. At this time, the first discriminator’s The input image includes the target domain image generated by the first generator and the target domain image acquired for training; the second generator uses the input target domain image to generate the source domain image, and the second discriminator is used to judge whether the input image is the second The source domain image generated by the generator. At this time, the input image of the second discriminator includes the source domain image generated by the second generator and the source domain image acquired for ...
Embodiment 2
[0111] The present invention also provides a training system for an image style transfer model, which is mainly implemented based on the method provided in the first embodiment above, as Figure 5 As shown, the system mainly includes:
[0112] The model construction and image data acquisition unit is used to construct an image style transfer model including two generators and two discriminators. A single generator and a single discriminator form an adversarial structure, which constitutes two adversarial structures, and obtains the used training source domain images and target domain images;
[0113] The total confrontation loss calculation unit is used to input both the source domain image and the target domain image to each confrontation structure, and calculate the total confrontation loss by using the output of the two confrontation structures;
[0114] The total cycle consistency loss calculation unit is used to input the output of the generator of the current confrontat...
PUM
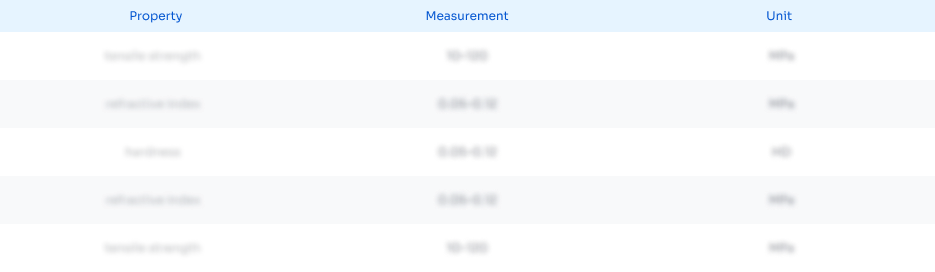
Abstract
Description
Claims
Application Information
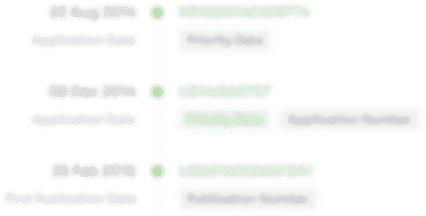
- R&D Engineer
- R&D Manager
- IP Professional
- Industry Leading Data Capabilities
- Powerful AI technology
- Patent DNA Extraction
Browse by: Latest US Patents, China's latest patents, Technical Efficacy Thesaurus, Application Domain, Technology Topic, Popular Technical Reports.
© 2024 PatSnap. All rights reserved.Legal|Privacy policy|Modern Slavery Act Transparency Statement|Sitemap|About US| Contact US: help@patsnap.com