Brain magnetic resonance image segmentation method based on scattering graph neural network
A magnetic resonance image and neural network technology, applied in the field of digital images, can solve the problems of high cost of segmentation and labeling, high time complexity, and inapplicability of 3D magnetic resonance images, so as to reduce the cost of labeling, reduce time complexity, and improve the model. The effect of segmentation efficiency
- Summary
- Abstract
- Description
- Claims
- Application Information
AI Technical Summary
Problems solved by technology
Method used
Image
Examples
Embodiment 1
[0051] A brain magnetic resonance image segmentation method based on scattergram neural network, such as figure 1 and figure 2 shown, including the following steps:
[0052] First, step S1: the method for extracting supervoxels is as follows: for the reference image and the image to be segmented, the fuzzy iterative clustering method is used to extract the supervoxels respectively, including the following five steps:
[0053] (1-1) uniformly sample N seed points in the brain region of the brain magnetic resonance image;
[0054] (1-2) Calculate the distance between each voxel and various sub-points in the brain magnetic resonance image, wherein the distance D(i, j) between the i-th voxel and the j-th seed point can be expressed as: D (i,j)=d I (i, j)+λd s (i, j), where d I (i, j) is the grayscale distance between the i-th voxel and the j-th seed point, which can be expressed as:
[0055] d I (i, j)=|I i -I j |
[0056] d s (i, j) is the spatial distance between the...
Embodiment
[0096] The following takes the MRBrainS18 data set data as an example to illustrate a brain magnetic resonance image segmentation method based on a scattergram neural network of the present invention.
PUM
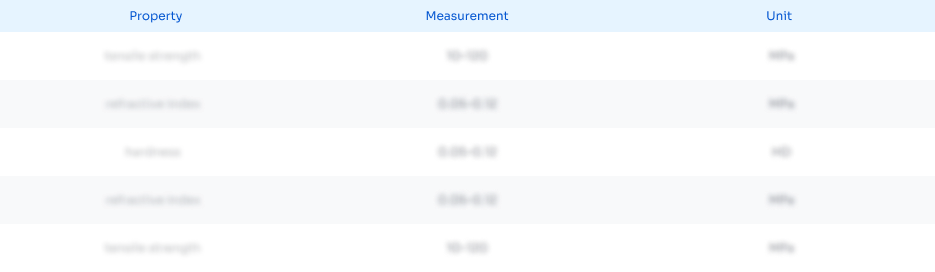
Abstract
Description
Claims
Application Information
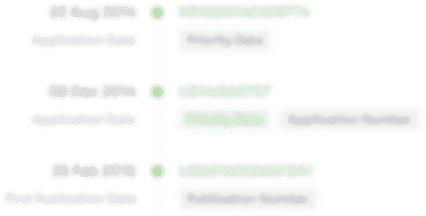
- R&D
- Intellectual Property
- Life Sciences
- Materials
- Tech Scout
- Unparalleled Data Quality
- Higher Quality Content
- 60% Fewer Hallucinations
Browse by: Latest US Patents, China's latest patents, Technical Efficacy Thesaurus, Application Domain, Technology Topic, Popular Technical Reports.
© 2025 PatSnap. All rights reserved.Legal|Privacy policy|Modern Slavery Act Transparency Statement|Sitemap|About US| Contact US: help@patsnap.com