A Few-Sample Defect Detection Method Based on Metric Learning
A metric learning and defect detection technology, applied in the field of defect detection of large-scale assembly parts, can solve the problems of low intelligence, long time consumption, inaccurate features, etc. Effect
- Summary
- Abstract
- Description
- Claims
- Application Information
AI Technical Summary
Problems solved by technology
Method used
Image
Examples
Embodiment Construction
[0047] The embodiments of the present invention will be described in further detail below with reference to the accompanying drawings.
[0048] see Figure 1-4, the present invention provides a small sample defect detection method based on metric learning, which specifically includes the following steps:
[0049] Step (1): Pass G 2 - GAN adversarial network for data augmentation of small sample defect datasets;
[0050] Step (2): Based on the self-adaptive convolutional neural network SKM-CNN of the convolution kernel, the features of the defect data set (auxiliary data set) similar to the small sample defect data set to be detected are extracted to generate a pre-training model;
[0051] Step (3): Migrate the pre-trained model to a few-shot defect detection network S based on metric learning 2 D 2 In N, the rapid identification and localization of defects is realized by the method of first target feature extraction and then metric learning.
[0052] In the step (1), thro...
PUM
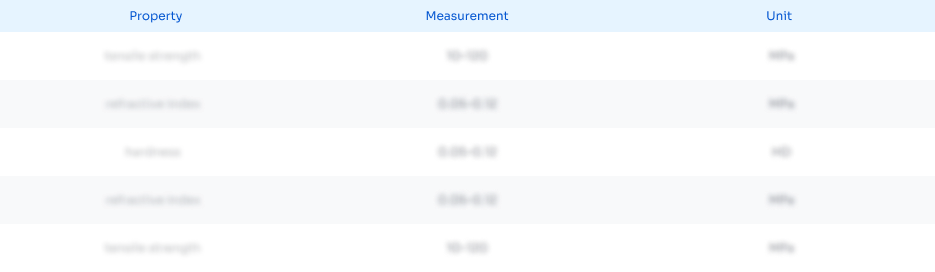
Abstract
Description
Claims
Application Information
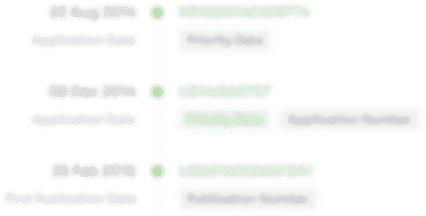
- R&D Engineer
- R&D Manager
- IP Professional
- Industry Leading Data Capabilities
- Powerful AI technology
- Patent DNA Extraction
Browse by: Latest US Patents, China's latest patents, Technical Efficacy Thesaurus, Application Domain, Technology Topic, Popular Technical Reports.
© 2024 PatSnap. All rights reserved.Legal|Privacy policy|Modern Slavery Act Transparency Statement|Sitemap|About US| Contact US: help@patsnap.com