Electrocardiosignal classification method and system based on multi-domain feature learning
A technology of ECG signal and classification method, which is applied in medical science, diagnosis, diagnostic recording/measurement, etc. It can solve the problems that the detection performance cannot meet the clinical application, and achieve the effect of improving effectiveness, precision and high accuracy
- Summary
- Abstract
- Description
- Claims
- Application Information
AI Technical Summary
Problems solved by technology
Method used
Image
Examples
Embodiment 1
[0056] In this embodiment, a classification network composed of a multi-channel feature extraction network layer, a feature fusion layer and a classification layer is constructed to identify the ECG signal during the onset of atrial fibrillation. The classification method of this embodiment mines the features corresponding to clinical diagnosis knowledge in the ECG data, and mines the features from different angles of the ECG data through the conversion of the one-dimensional ECG data in different domains, so as to realize automatic learning under the guidance of medical knowledge , and finally fused a variety of features to construct a classification layer that can accurately identify patients with atrial fibrillation. The classification result of the present invention has important significance and application value for assisting doctors in clinical decision-making. The specific implementation process of the classification method of the present invention includes the followi...
Embodiment 2
[0099] This embodiment provides a system based on the ECG signal classification method, which includes: a preprocessing module, a multi-domain feature extraction module, a fusion module, a classification module, and a training module.
[0100] Wherein, the preprocessing module is used to preprocess the ECG signal, the preprocessing at least includes: extracting the RR interval sequence and P wave region data of the ECG signal, and performing time-frequency conversion on the P wave region data to obtain Time-frequency diagram of the P-wave region.
[0101] The multi-domain feature extraction module is used to perform multi-domain feature extraction on ECG signals based on the multi-channel feature extraction network layer. The multi-channel feature extraction network layer includes a time-series feature extraction network for heart rhythm feature channels, a non-time-series feature extraction network for atrial feature channels, and a global feature extraction network. The mul...
Embodiment 3
[0116] This embodiment provides an electronic terminal, which includes one or more processors; and one or more memories; the processor invokes a computer program stored in the memory to execute: an electrocardiogram based on multi-domain feature learning Steps of a signal classification method.
PUM
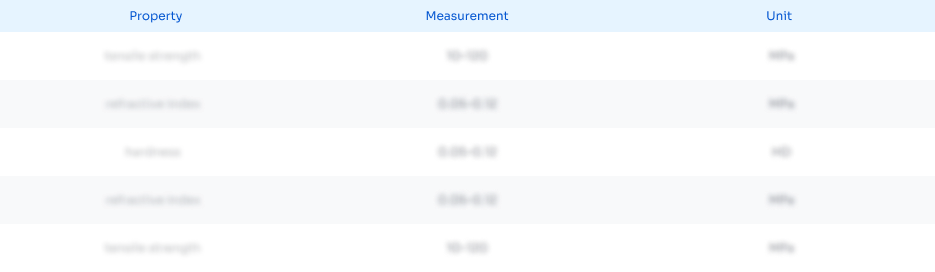
Abstract
Description
Claims
Application Information
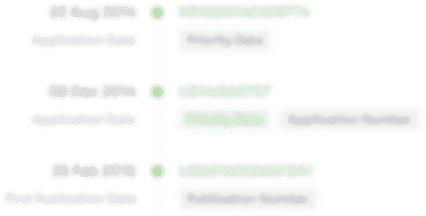
- R&D
- Intellectual Property
- Life Sciences
- Materials
- Tech Scout
- Unparalleled Data Quality
- Higher Quality Content
- 60% Fewer Hallucinations
Browse by: Latest US Patents, China's latest patents, Technical Efficacy Thesaurus, Application Domain, Technology Topic, Popular Technical Reports.
© 2025 PatSnap. All rights reserved.Legal|Privacy policy|Modern Slavery Act Transparency Statement|Sitemap|About US| Contact US: help@patsnap.com