Mass data-based causal group extraction method and system, and computer readable storage medium
An extraction method and mass data technology, applied in reasoning methods, computer components, calculations, etc., to achieve high reliability, improve accuracy, and reduce noise data
- Summary
- Abstract
- Description
- Claims
- Application Information
AI Technical Summary
Problems solved by technology
Method used
Image
Examples
Embodiment 1
[0036] like figure 1 As shown, a first aspect of the present invention provides a method for extracting causal groups based on massive data, comprising the following steps:
[0037] S1: Obtain network texts and store them separately by time period;
[0038] It should be noted that the network text obtained in the embodiments of the present application is the content disclosed on the Internet. Hadoop distributed storage system HDFS.
[0039] S2: uniformly sample the acquired network text to obtain a sample set and pre-label the sample set;
[0040] The obtained Internet samples are uniformly sampled to obtain a sample set. It should be noted that the number of samples in the sample set should not be too small. In a specific implementation, the number of samples can be about 10,000. Then the sample set is pre-labeled. Pre-labeling is to use the method of keyword and regular matching to mark whether each sample contains causal relationship for the first time. For example, reg...
Embodiment 2
[0059] A second aspect of the present invention provides a system for extracting causal groups based on massive data. The system includes: a memory and a processor, wherein the memory includes a method program for extracting causal groups based on massive data. When the event group extraction method program is executed by the processor, the following steps are implemented:
[0060] S1: Obtain network texts and store them separately by time period;
[0061] It should be noted that the network text obtained in the embodiments of the present application is the content disclosed on the Internet. Hadoop distributed storage system HDFS.
[0062] S2: uniformly sample the acquired network text to obtain a sample set and pre-label the sample set;
[0063] The obtained Internet samples are uniformly sampled to obtain a sample set. It should be noted that the number of samples in the sample set should not be too small. In a specific implementation, the number of samples can be about 10...
Embodiment 3
[0084] This embodiment illustrates the method of the present invention by processing specific triples. For example, in a specific embodiment, the above-mentioned BERT+CRF model is used to perform causal extraction on network text to obtain the following triples:
[0085] [("Cool down by 5 degrees today", 0.55,"It will rain tomorrow"),("Cold wave is coming",0.9,"Down jacket sales increase"),("Wire short circuit",0.7,"Fire broke out"),( "Double Eleven is Coming", 0.65, "The manufacturer's down jacket is out of stock"), ("Cable short circuit", 0.55, "Cause a fire") ("Cold air goes south", 0.85, "Down jacket hot sale"), ("Temperature Dip", 0.55, "Down jacket in short supply"), ("Aging line", 0.9, "Fire hazard"), ("Down jacket in short supply", 0.55, "Temperature drop")]
[0086] Calculate the semantic vector of the above triplet, use the semantic vector to calculate the cosine distance, and use the cosine distance (cosine distance = 1-cosine similarity) as the metric index to clus...
PUM
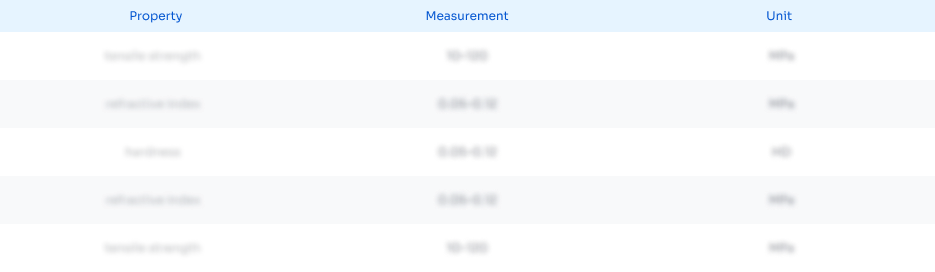
Abstract
Description
Claims
Application Information
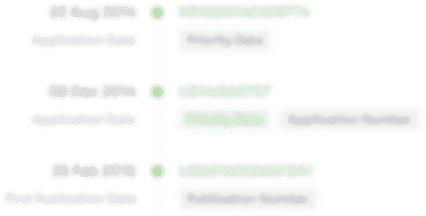
- R&D Engineer
- R&D Manager
- IP Professional
- Industry Leading Data Capabilities
- Powerful AI technology
- Patent DNA Extraction
Browse by: Latest US Patents, China's latest patents, Technical Efficacy Thesaurus, Application Domain, Technology Topic, Popular Technical Reports.
© 2024 PatSnap. All rights reserved.Legal|Privacy policy|Modern Slavery Act Transparency Statement|Sitemap|About US| Contact US: help@patsnap.com