Event sequence prediction method based on time sequence convolution and relation modeling
A technology of event sequences and prediction methods, applied in prediction, neural learning methods, character and pattern recognition, etc., can solve problems such as low computational efficiency, limited model performance, no event mining and analysis, etc., to achieve rich expression ability and improve prediction The effect of improving performance and computing efficiency
- Summary
- Abstract
- Description
- Claims
- Application Information
AI Technical Summary
Problems solved by technology
Method used
Image
Examples
Embodiment
[0099] In order to verify the effectiveness of the present invention, we conduct example verification on two event sequence prediction task datasets collected in real scenarios, including IPTV dataset and MIMIC-II dataset. Among them, the IPTV dataset is the event sequence data provided by China Telecom Corporation on the behavior of users watching cable TV programs, including the viewing behavior sequences of 2,967 users; the MIMIC-II dataset is the medical diagnosis-related event sequence data of patients provided by the medical center. Visit data for 53,423 patients who visited the medical center between 2001 and 2008 were recorded. The present embodiment now takes an event sequence data for testing in the IPTV data set as an example, and predicts events according to the following steps:
[0100] 1. Perform data preprocessing on the event sequence data, including data cleaning and calculation of interval time. The data cleaning process includes the removal of invalid sampl...
PUM
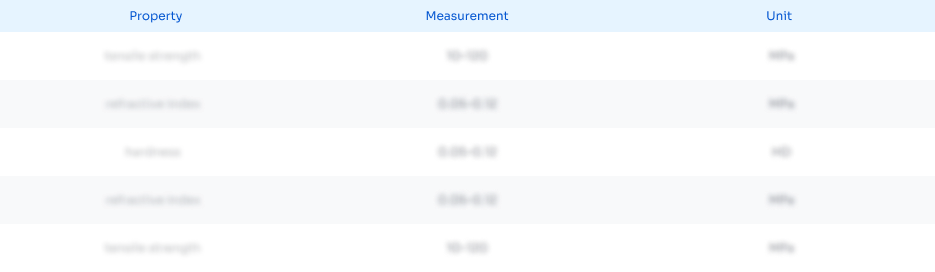
Abstract
Description
Claims
Application Information
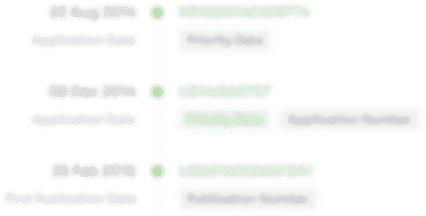
- R&D
- Intellectual Property
- Life Sciences
- Materials
- Tech Scout
- Unparalleled Data Quality
- Higher Quality Content
- 60% Fewer Hallucinations
Browse by: Latest US Patents, China's latest patents, Technical Efficacy Thesaurus, Application Domain, Technology Topic, Popular Technical Reports.
© 2025 PatSnap. All rights reserved.Legal|Privacy policy|Modern Slavery Act Transparency Statement|Sitemap|About US| Contact US: help@patsnap.com