Edge end time series prediction method based on improved knowledge distillation ESN
A technology of time series and forecasting methods, applied in forecasting, neural learning methods, data processing applications, etc., can solve problems such as limited forecasting performance, insufficient long-term memory ability, and reduced ESN computing overhead, so as to improve long-term memory ability and reduce computing costs. Overhead, the effect of optimizing the structure of the reserve pool
- Summary
- Abstract
- Description
- Claims
- Application Information
AI Technical Summary
Problems solved by technology
Method used
Image
Examples
Embodiment Construction
[0075] The technical solutions of the present invention will be further described in detail below with reference to the accompanying drawings.
[0076] Edge-side time series prediction method based on improved Knowledge Distillation (KD) Echo State Network (ESN), refer to figure 1 ,Specific steps are as follows:
[0077] Step 1: Construct a time series prediction model based on improved knowledge distillation ESN, such as figure 2 shown. Specific steps are as follows:
[0078] Step 1-1: Take LSTM as the teacher network and set its parameters. LSTM is responsible for teaching long-term memory ability. It consists of input layer, hidden layer, and output layer. The hidden layer adopts a double-layer structure; the input layer and the hidden layer, the hidden layer and the hidden layer, and the output layer and the hidden layer are fully connected; set The number of neurons in the input layer and output layer of LSTM is K and L respectively; the number of neurons in the firs...
PUM
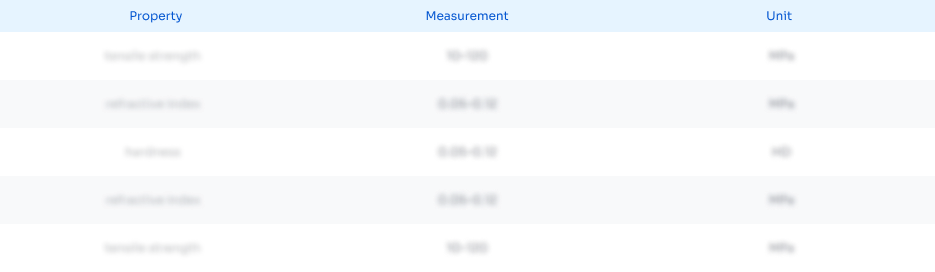
Abstract
Description
Claims
Application Information
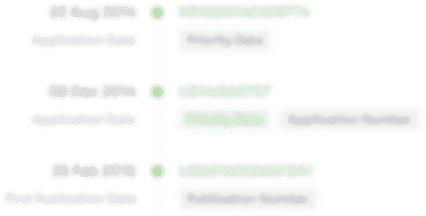
- Generate Ideas
- Intellectual Property
- Life Sciences
- Materials
- Tech Scout
- Unparalleled Data Quality
- Higher Quality Content
- 60% Fewer Hallucinations
Browse by: Latest US Patents, China's latest patents, Technical Efficacy Thesaurus, Application Domain, Technology Topic, Popular Technical Reports.
© 2025 PatSnap. All rights reserved.Legal|Privacy policy|Modern Slavery Act Transparency Statement|Sitemap|About US| Contact US: help@patsnap.com