Text entity relationship classification method based on small sample learning
A technology of entity relationship and classification method, which is applied in the field of text data recognition, can solve the problems of classification result deviation, relationship classification effect influence, prototype deviation and other problems, and achieve the effect of eliminating error, improving operation speed and solving the problem of feature sparsity
- Summary
- Abstract
- Description
- Claims
- Application Information
AI Technical Summary
Problems solved by technology
Method used
Image
Examples
Embodiment Construction
[0043] Below in conjunction with specific embodiments, the present invention will be further illustrated, and it should be understood that these embodiments are only used to illustrate the present invention and not to limit the scope of the present invention. The modifications all fall within the scope defined by the appended claims of this application.
[0044] like figure 1 As shown, the text entity relationship classification method based on few-shot learning includes the following steps:
[0045] Step 1 Given a data set, including support set sentences and query set sentences, use CNN network as an instance encoder to encode the support set sentences and query set sentences, and convert them into low-dimensional instance vectors, so as to obtain the extracted corpus. Entity pair feature. The specific process is:
[0046] 1-1 Select the FewRel dataset that is used more in the field of small sample relation classification;
[0047] 1-2 Predefine a set of relation types f...
PUM
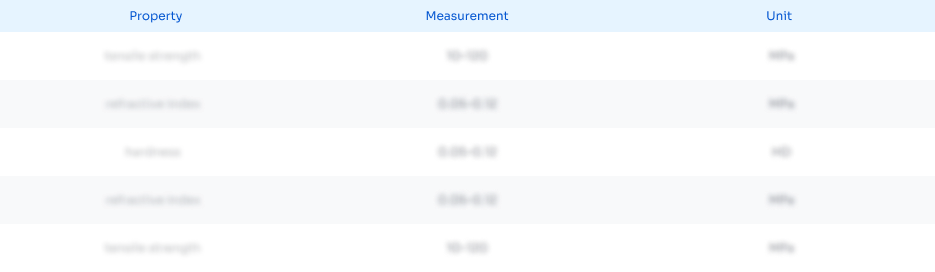
Abstract
Description
Claims
Application Information
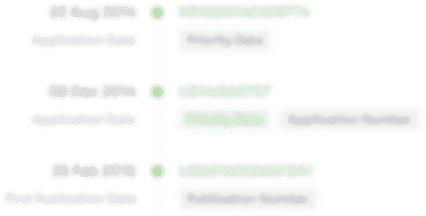
- R&D Engineer
- R&D Manager
- IP Professional
- Industry Leading Data Capabilities
- Powerful AI technology
- Patent DNA Extraction
Browse by: Latest US Patents, China's latest patents, Technical Efficacy Thesaurus, Application Domain, Technology Topic, Popular Technical Reports.
© 2024 PatSnap. All rights reserved.Legal|Privacy policy|Modern Slavery Act Transparency Statement|Sitemap|About US| Contact US: help@patsnap.com