Traffic scene semantic segmentation method based on boundary-guided context aggregation
A traffic scene and semantic segmentation technology, applied in the field of image processing, can solve the problems of difficult semantic segmentation tasks and wrong boundary estimation, and achieve the effect of improving segmentation performance and strong robustness.
- Summary
- Abstract
- Description
- Claims
- Application Information
AI Technical Summary
Problems solved by technology
Method used
Image
Examples
Embodiment 1
[0054] figure 1 Shown is a flowchart of a traffic scene semantic segmentation method for boundary-guided context aggregation according to an embodiment of the present invention, and the specific steps are as follows:
[0055] Step 1, obtain a traffic scene image.
[0056] Obtain the public dataset of traffic scenes and the corresponding segmentation labels.
[0057] Step 2, perform data processing on the traffic scene image.
[0058] (2-a) Synchronously flip the image in the original sample data and the corresponding segmentation label horizontally;
[0059] (2-b) Scale the image obtained in step (2-a) and the corresponding segmentation label to m 1 ×m 2 pixel size, where m 1 and m 2 are the width and height of the zoomed image, respectively, in this embodiment, m is preferred 1 is 769, m 2 is 769;
[0060] (2-c) Normalize the image obtained by scaling in step (2-b) and the corresponding segmentation label to form a processed sample data set.
[0061] Step 3, build a...
Embodiment 2
[0091] The method in Example 1 is used to conduct a traffic scene image semantic segmentation experiment on the public data set. There are 19 categories in the dataset, namely road, sidewalk, building, wall, fence, pole, traffic light, traffic Signal (traffic sign), vegetation (vegetation), terrain (terrain), sky (sky), pedestrian (person), rider (rider), car (car), truck (truck), bus (bus), train (train) ), motorcycles and bicycles. The experimental operating system is Linux, which is implemented based on the PyTorch1.6.0 framework of CUDA10.0 and cuDNN7.6.0, and uses 4 pieces of NVIDIA GeForce RTX 2080Ti (11GB) hardware.
[0092] This embodiment uses the intersection-over-union (IoU) index to compare the six methods of RefineNet, PSPNet, AAF, PSANet, AttaNet, DenseASPP and the present invention on the test set. The average result of this index on all categories is expressed by mIoU, Calculated as follows:
[0093]
[0094] K+1 represents the total number of categories ...
PUM
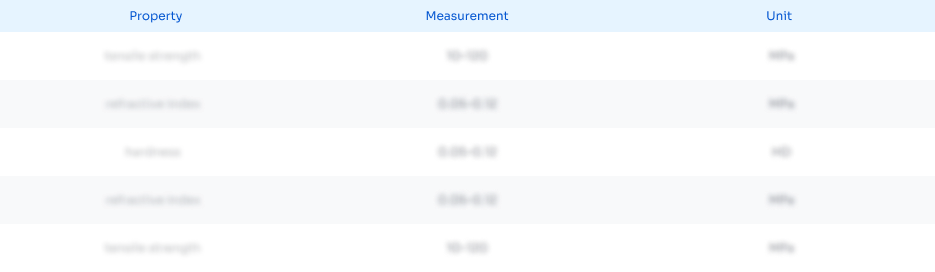
Abstract
Description
Claims
Application Information
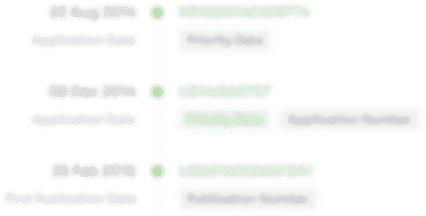
- R&D
- Intellectual Property
- Life Sciences
- Materials
- Tech Scout
- Unparalleled Data Quality
- Higher Quality Content
- 60% Fewer Hallucinations
Browse by: Latest US Patents, China's latest patents, Technical Efficacy Thesaurus, Application Domain, Technology Topic, Popular Technical Reports.
© 2025 PatSnap. All rights reserved.Legal|Privacy policy|Modern Slavery Act Transparency Statement|Sitemap|About US| Contact US: help@patsnap.com