Language model compression method based on uncertainty estimation knowledge distillation
An uncertainty and language model technology, applied in the field of compression of pre-trained language models, can solve problems such as low network compression rate, low efficiency, and large computational burden, and achieve the goals of reducing the number of parameters, improving training efficiency, and improving reasoning performance Effect
- Summary
- Abstract
- Description
- Claims
- Application Information
AI Technical Summary
Problems solved by technology
Method used
Image
Examples
Embodiment Construction
[0031] The present invention will be described in further detail below with reference to the accompanying drawings and specific embodiments.
[0032] refer to figure 1 , a pre-trained language model compression method based on uncertainty estimation knowledge distillation, the implementation steps are as follows:
[0033] Step 1. Obtain training and testing datasets.
[0034] Obtain the data set in GLUE, the basic task of public natural language understanding. The data set contains various tasks of common natural language processing, which can better test the comprehensive performance of the language model.
[0035] This example is obtained from the following four types of data sets in this data set, and subsequent experimental test tasks are performed:
[0036] First, the language acceptability corpus CoLA is a single-sentence classification task, and its corpus comes from language theory books and journals, where each word sequence is marked as grammatical;
[0037] Secon...
PUM
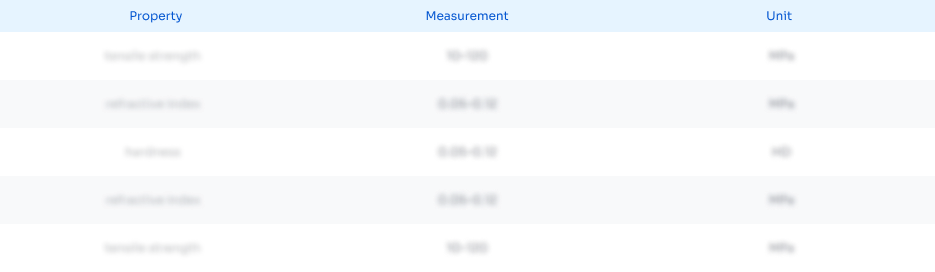
Abstract
Description
Claims
Application Information
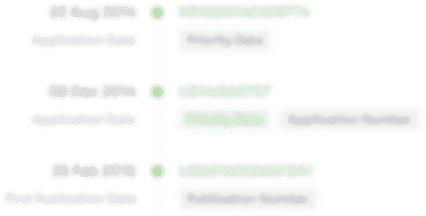
- R&D Engineer
- R&D Manager
- IP Professional
- Industry Leading Data Capabilities
- Powerful AI technology
- Patent DNA Extraction
Browse by: Latest US Patents, China's latest patents, Technical Efficacy Thesaurus, Application Domain, Technology Topic, Popular Technical Reports.
© 2024 PatSnap. All rights reserved.Legal|Privacy policy|Modern Slavery Act Transparency Statement|Sitemap|About US| Contact US: help@patsnap.com