Physical layer deception detection method based on deep reinforcement learning
A technology of reinforcement learning and deception detection, applied in neural learning methods, biological neural network models, electrical components, etc., to achieve fast and accurate detection and dynamic continuous selection.
- Summary
- Abstract
- Description
- Claims
- Application Information
AI Technical Summary
Problems solved by technology
Method used
Image
Examples
Embodiment 1
[0038] Example 1: see figure 1 , figure 2 , a physical layer deception detection method based on deep reinforcement learning, including the following steps:
[0039] Step 1. Establish a spoofing attack scenario, and the receiver extracts the physical layer channel state information between the sender and the receiver to represent the physical layer fingerprint feature;
[0040] Step 2. Build a binary hypothesis testing model;
[0041] Step 3, constructing the state value with the dynamic physical layer fingerprint feature, selecting and constructing the behavior value with the threshold value, using the Bayesian risk function as the instantaneous benefit function, and establishing the state-behavior-benefit triplet;
[0042] Step 4. Based on the deep deterministic policy gradient framework, a dynamic selection method of detection threshold is designed to detect physical layer spoofing attacks.
[0043] The spoofing attack scenario established in step 1 is that Alice and Bo...
Embodiment 2
[0067] Example 2: as figure 1 As shown, it is a schematic diagram of a spoofing attack scenario. The present invention proposes a physical layer spoofing detection method based on deep reinforcement learning. The method specifically includes the following steps:
[0068] Step 1. Establish a spoofing attack scenario. Alice and Bob are legitimate users, Alice is the transmitter, Bob is the receiver, and Eve is the spoofed user; it is assumed that the distance between the spoofed attacker and the legitimate transmitter and legitimate receiver is greater than half a distance. Wavelength, that is, the illegal channel is not related to the legal channel; the spoofing attack is an active attack, that is, the spoofing attacker and the legitimate transmitter do not transmit signals at the same time, and the attack probability of Eve pretending to be Alice to send a spoofing signal to Bob is y∈[0,Y MAX ), where the maximum attack probability Y MAX <1. The physical layer channel inform...
PUM
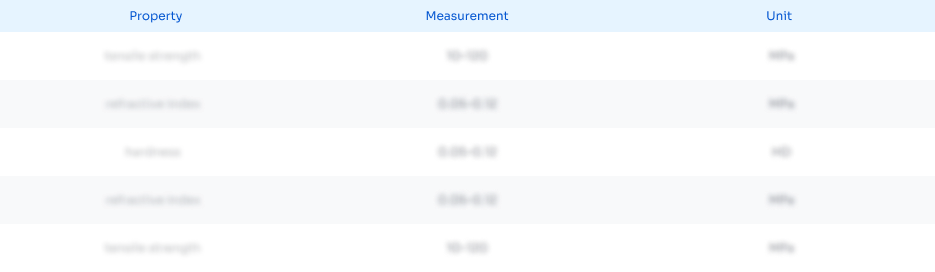
Abstract
Description
Claims
Application Information
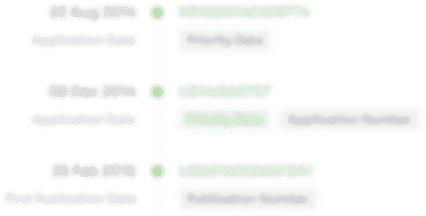
- R&D Engineer
- R&D Manager
- IP Professional
- Industry Leading Data Capabilities
- Powerful AI technology
- Patent DNA Extraction
Browse by: Latest US Patents, China's latest patents, Technical Efficacy Thesaurus, Application Domain, Technology Topic, Popular Technical Reports.
© 2024 PatSnap. All rights reserved.Legal|Privacy policy|Modern Slavery Act Transparency Statement|Sitemap|About US| Contact US: help@patsnap.com