Encrypted traffic identification and classification method and system based on direct push graph
A technology of traffic identification and classification method, which is applied in the field of encrypted traffic identification and classification method and system based on transductive graph, which can solve the problem that intelligent encrypted traffic identification and classification technology cannot be well applied in actual deployment, is susceptible to interference, and encrypted traffic identification Problems such as the inability of the classification model to achieve stable recognition and classification effects
- Summary
- Abstract
- Description
- Claims
- Application Information
AI Technical Summary
Problems solved by technology
Method used
Image
Examples
example 1
[0064] Example 1 Using the encrypted traffic identification and classification algorithm based on the direct inference graph to identify and classify encrypted traffic under unknown networks
[0065] Taking the independently collected dataset CrossNet2022 as the dataset, the CrossNet2022 dataset contains encrypted traffic data collected in two network environments. For each network environment, the encrypted traffic data generated by the same network application is collected. These traffics are generated from 20 commonly used web application:
[0066] 1) First, for the encrypted traffic data of 20 network applications collected in one of the network environments, use the five-tuple of {destination IP, destination port, source IP, source port, transport layer protocol} as the key to segment the network session. . Divide the collected set of raw packets into individual network sessions;
[0067] 2) extract the destination IP address of the network layer of each network session...
example 2
[0079] Example 2 Identifying and classifying a new class of encrypted traffic for network applications not included in the training set using a transductive graph-based encrypted traffic identification and classification algorithm
[0080] Use two sub-data sets of CrossNet2022 as instance samples, in which 15 classes in CrossNet2022_A are used as training sets, and their labels are known; the data in CrossNet2022_B are used as test sets, including all 20 classes, which need to be identified that are not included in CrossNet2022_A 5 categories.
[0081] 1) Repeat 1)-9) in Example 1;
[0082] 2) For nodes to be tested that have not obtained predicted class labels in the transduction graph, the network sessions included in them are determined as new classes, that is, encrypted traffic sessions generated by network applications that do not appear in the training set.
[0083] Comparing the results of the method of the present invention and other methods, all methods are initializ...
PUM
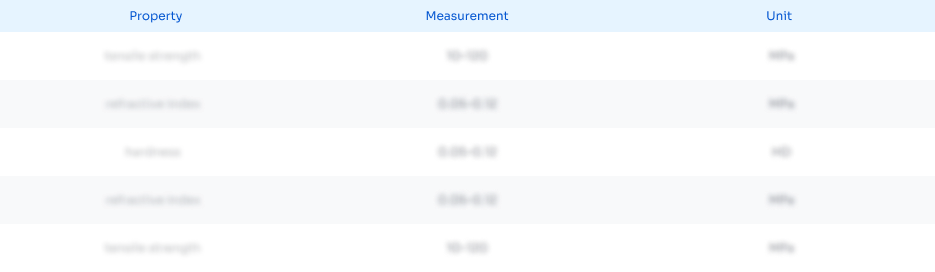
Abstract
Description
Claims
Application Information
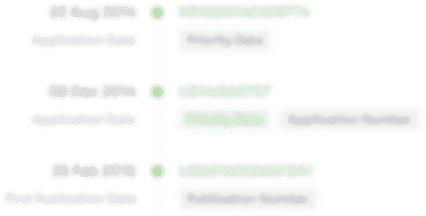
- R&D Engineer
- R&D Manager
- IP Professional
- Industry Leading Data Capabilities
- Powerful AI technology
- Patent DNA Extraction
Browse by: Latest US Patents, China's latest patents, Technical Efficacy Thesaurus, Application Domain, Technology Topic.
© 2024 PatSnap. All rights reserved.Legal|Privacy policy|Modern Slavery Act Transparency Statement|Sitemap