ICU (Intensive Care Unit) hospitalization duration and death risk prediction method based on convolutional neural network
A convolutional neural network, risk prediction technology, applied in the field of ICU length of stay and mortality risk prediction based on convolutional neural network, can solve the problems of poor performance, poor prediction accuracy, poor effect and so on
- Summary
- Abstract
- Description
- Claims
- Application Information
AI Technical Summary
Problems solved by technology
Method used
Image
Examples
Embodiment Construction
[0063] figure 1 A schematic flowchart of the method of the method of the present invention: this convolutional neural network-based ICU length of stay and mortality risk prediction method provided by the present invention includes the following steps:
[0064] S1. Obtain basic data from an existing electronic medical record database, process and classify it, and obtain a training data set, a verification data set and a test data set; the specific steps include the following:
[0065] For the ICU length of stay prediction task, obtain the data of the remaining length of stay of the inpatients in each hour during the stay in the ICU; and only obtain the data of X days after hospitalization; X is a set integer value;
[0066] For the mortality risk prediction task, only the first 24 hours of ICU inpatient data were obtained after ICU admission;
[0067] Obtain clinical time series, static demographic data and diagnostic data of inpatients;
[0068] The clinical time series incl...
PUM
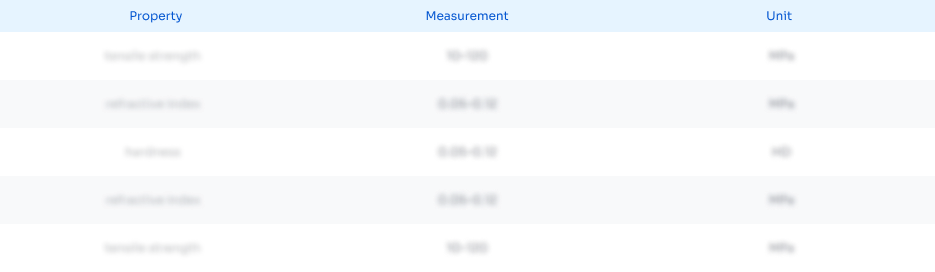
Abstract
Description
Claims
Application Information
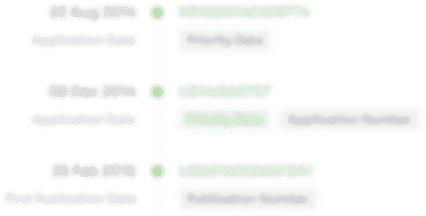
- R&D Engineer
- R&D Manager
- IP Professional
- Industry Leading Data Capabilities
- Powerful AI technology
- Patent DNA Extraction
Browse by: Latest US Patents, China's latest patents, Technical Efficacy Thesaurus, Application Domain, Technology Topic.
© 2024 PatSnap. All rights reserved.Legal|Privacy policy|Modern Slavery Act Transparency Statement|Sitemap