Classification and identification method for communication signal modulating mode based on ART2A-DWNN
A technology of ART2A and modulation mode, which is applied in the direction of character and pattern recognition, modulated carrier system, neural learning method, etc., can solve the problems of long classification recognition judgment cycle and low recognition accuracy, and achieve good self-adaptive learning and self-categorization Capability, simple calculation process, and good scalability
- Summary
- Abstract
- Description
- Claims
- Application Information
AI Technical Summary
Problems solved by technology
Method used
Image
Examples
specific Embodiment approach 1
[0026] Specific implementation mode one: the following combination Figure 1 to Figure 5 Describe this embodiment, this embodiment is realized based on ART2A neural network and DWNN neural network, ART2A neural network is made up of attention subsystem, orientation subsystem and modulation type secondary judgment module, attention subsystem is represented by short-term memory characteristic field F 1 and short-term memory category representation field F 2 composition, the warning threshold ρ is pre-stored in the orientation subsystem, and the classification and identification process of its communication signal modulation mode is:
[0027] Step 1. Extract the eigenvector of the communication signal, use the extracted eigenvector as the input vector of the ART2A neural network, and apply the ART2A-E algorithm to process the input vector of the ART2A neural network: set the input vector as an N-dimensional column vector X(k), input the N-dimensional column vector X(k) to the sh...
specific Embodiment approach 2
[0041] Specific implementation mode two: this implementation mode is a further description of step two in the first implementation mode: the first winning label j in the above step two 1 * (k) is calculated as:
[0042] j 1 * (k)=arg(min[||X(k)-W j*(k) (k)||]), j=1~μ(k),
[0043] Where: ||X(k)-W j*(k) (k)|| means X(k) and W j*(k) the Euclidean distance of (k),
[0044] X(k) and W j*(k) The formula for calculating the Euclidean distance of (k) is:
[0045] | | X ( k ) - W j * ( k ) ( k ) | | = [ Σ i = 1 N ...
specific Embodiment approach 3
[0048] Specific embodiment three: this embodiment is a further description of step three in embodiment one: in step three, the second judgment module of the modulation type determines the vector Y(k) output after clustering as L-type modulation type MT i The modulation method is: the class L modulation type MT i Set the corresponding warning threshold value for each type of modulation type, according to the warning threshold value, one or more continuous output nodes [Y i (k),...,Y j (k)] T Decision as a modulation type MT i , where 1≤i≤j≤M.
PUM
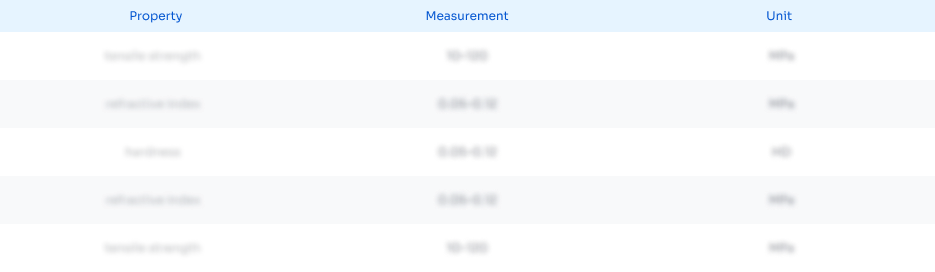
Abstract
Description
Claims
Application Information
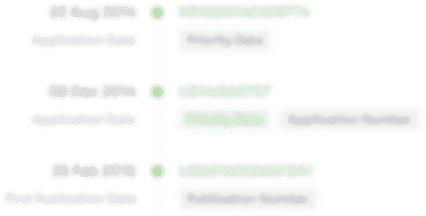
- R&D Engineer
- R&D Manager
- IP Professional
- Industry Leading Data Capabilities
- Powerful AI technology
- Patent DNA Extraction
Browse by: Latest US Patents, China's latest patents, Technical Efficacy Thesaurus, Application Domain, Technology Topic.
© 2024 PatSnap. All rights reserved.Legal|Privacy policy|Modern Slavery Act Transparency Statement|Sitemap