Unsupervised cluster characteristic selection method based on Laplace regularization
A feature selection and Laplacian matrix technology, applied in the field of data processing, can solve problems such as data complexity, dimensional disaster practicality, and affecting learning efficiency and effect
- Summary
- Abstract
- Description
- Claims
- Application Information
AI Technical Summary
Problems solved by technology
Method used
Image
Examples
Embodiment Construction
[0053] In order to describe the present invention more specifically, the clustering method of the present invention will be described in detail below in conjunction with the drawings and specific embodiments.
[0054] Such as figure 1 As shown, an unsupervised clustering feature selection method based on Laplacian regularization includes the following steps:
[0055] (1) Construct the sample feature matrix.
[0056] In this embodiment, the ORL face data set is taken as an example, and the statistical information of the data set is shown in Table 1.
[0057] Table 1
[0058] data set
Face image frame number
Number of face categories
number of image features
ORL
1400
20
1024
[0059] Among them, there are 1400 frames of face images in the ORL face dataset, and the 1400 frames of face images are composed of 20 face images of people with different appearances (70 frames of face images for each person).
[0060] Select five ...
PUM
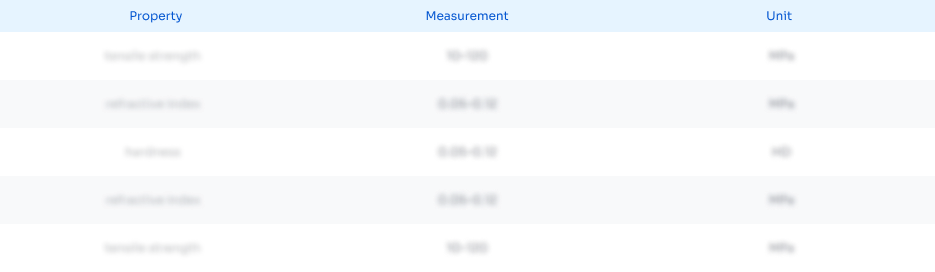
Abstract
Description
Claims
Application Information
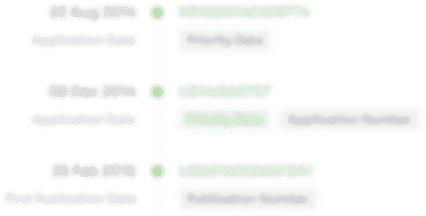
- R&D
- Intellectual Property
- Life Sciences
- Materials
- Tech Scout
- Unparalleled Data Quality
- Higher Quality Content
- 60% Fewer Hallucinations
Browse by: Latest US Patents, China's latest patents, Technical Efficacy Thesaurus, Application Domain, Technology Topic, Popular Technical Reports.
© 2025 PatSnap. All rights reserved.Legal|Privacy policy|Modern Slavery Act Transparency Statement|Sitemap|About US| Contact US: help@patsnap.com