Sewage-disposal soft measurement method on basis of integrated neural network
A neural network, sewage treatment technology, applied in biological neural network models, testing water, material inspection products, etc., can solve the problem of output accuracy, the problem of neural network learning algorithm selection and other issues
- Summary
- Abstract
- Description
- Claims
- Application Information
AI Technical Summary
Problems solved by technology
Method used
Image
Examples
Embodiment Construction
[0091] The experimental data comes from the daily report of influent and effluent water quality of a small sewage treatment plant. Use the integrated neural network to establish the soft sensor model, the input parameters are Q, SS, DO, MLSS, PH, NH 4 -N, NO 3 -, ORP, and influent turbidity, the output parameters are effluent COD, effluent BOD, and effluent TN. The neural network structure is as follows figure 1 , there are 24 groups of training samples and 8 groups of prediction samples.
[0092] The specific steps of neural network prediction are as follows:
[0093] (1). Create an integrated neural network;
[0094] Specific steps are as follows:
[0095] ①. Create a sub-neural network: the network includes three sub-feedforward neural networks, the number of layers of the network is three layers, and the input auxiliary variables of the three sub-neural networks are influent flow Q, influent SS, aeration tank DO, MLSS and Influent flow Q, aeration tank DO, MLSS, PH,...
PUM
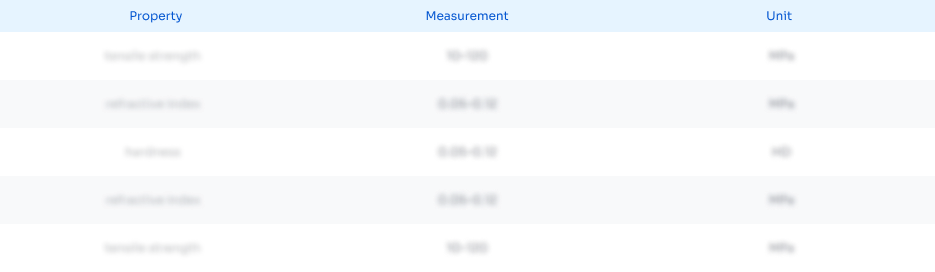
Abstract
Description
Claims
Application Information
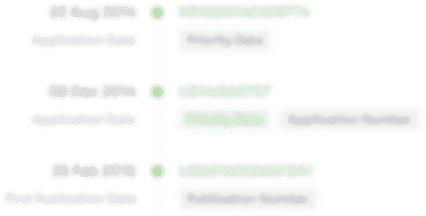
- R&D
- Intellectual Property
- Life Sciences
- Materials
- Tech Scout
- Unparalleled Data Quality
- Higher Quality Content
- 60% Fewer Hallucinations
Browse by: Latest US Patents, China's latest patents, Technical Efficacy Thesaurus, Application Domain, Technology Topic, Popular Technical Reports.
© 2025 PatSnap. All rights reserved.Legal|Privacy policy|Modern Slavery Act Transparency Statement|Sitemap|About US| Contact US: help@patsnap.com