Remaining Life Prediction Method for Monotone Echo State Networks
An echo state network and life prediction technology, applied in special data processing applications, instruments, electrical digital data processing, etc., can solve problems such as low computing efficiency, poor approximation performance, and inability to accurately predict lifespan, etc., to improve approximation performance, improve The effect of approximating efficiency and narrowing the scope of optimization
- Summary
- Abstract
- Description
- Claims
- Application Information
AI Technical Summary
Problems solved by technology
Method used
Image
Examples
specific Embodiment approach 1
[0028] Specific implementation mode one: the following combination figure 2 Describe this embodiment, the method for predicting the remaining life of the monotone echo state network described in this embodiment, step 1, randomly establish the monotone echo state network model MONESN:
[0029] The monotone echo state network model MONESN includes an input unit u(n), an internal processing unit x(n) and an output unit y(n), where n is the moment of the corresponding system state transition; the input unit collects simulated physical parameters of the turbine engine, including temperature , pressure and speed parameters of the system; or the input unit collects the charge and discharge data of the lithium-ion battery;
[0030] u(n) represents the value of the input unit at time n, x(n) represents the value of the internal processing unit at time n, and y(n) represents the value of the output unit at time n.
[0031] The output unit outputs the number of remaining operating cycl...
specific Embodiment approach 2
[0039] Specific implementation mode two: this implementation mode further explains implementation mode one, and the monotone echo state network model MONESN randomly established in step one includes an input unit u(n), an internal processing unit x(n) and an output unit y(n),
[0040] u(n)=(u 1 (n), u 1 (n),...,u j (n),...,u L (n)),j=1,2,...,L;
[0041] x(n)=(x 1 (n), x 2 (n),...,x s (n),...,x N (n)), s=1,2,...,N;
[0042] y(n)=(y 1 (n),y 2 (n),...,y i (n),...,y M (n)), i=1,2,...,M;
[0043] n is the moment corresponding to the state transition of the system, L, M and N are all positive integers;
[0044] The process of establishing MONESN is:
[0045] Step 11. Randomly establish an N×L-dimensional input weight matrix W in , N×N-dimensional internal connection weight matrix W 0 and N×M dimensional feedback weight matrix W back ;
[0046] Step 12, according to the formula:
[0047] W 1 =W 0 / |λ max |
[0048] get W 1 , where |λ max | is W 0 The absolut...
specific Embodiment approach 3
[0054] Specific implementation mode three: this implementation mode further explains implementation mode one, the process of carrying out network dynamic training to the untrained MONESN that step one is set up described in step two is:
[0055] Step 21, initialize the internal processing unit x(0)=0, output unit y(0)=0;
[0056] Step 22, input sequence u j (n) and the real output sample sequence y i (n) Drive MONESN and update the equation according to the internal processing unit of MONESN
[0057] x(n)=f(W in u(n)+Wx(n-1)+W back y(n-1))
[0058] and
[0059] y(n)=W out (u(n),x(n))
[0060] Get the internal neuron state at each moment;
[0061] Store the input and internal state at each moment in the form of row vectors in the T×(L+N)-dimensional internal state matrix C; and store the real output at the corresponding moment in the form of row vectors in T×M as the output matrix d;
[0062] Step two and three, constructing constraint inequality: the transposition of...
PUM
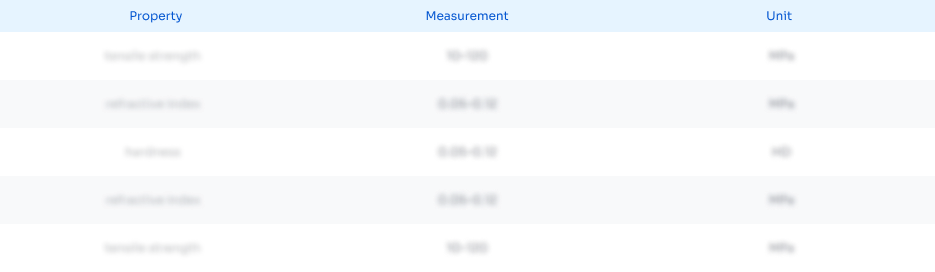
Abstract
Description
Claims
Application Information
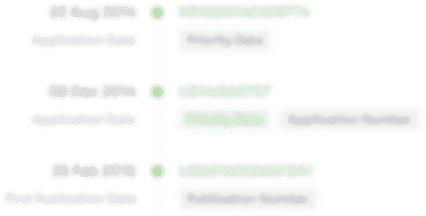
- R&D Engineer
- R&D Manager
- IP Professional
- Industry Leading Data Capabilities
- Powerful AI technology
- Patent DNA Extraction
Browse by: Latest US Patents, China's latest patents, Technical Efficacy Thesaurus, Application Domain, Technology Topic, Popular Technical Reports.
© 2024 PatSnap. All rights reserved.Legal|Privacy policy|Modern Slavery Act Transparency Statement|Sitemap|About US| Contact US: help@patsnap.com