Maximum average entropy-based scale-invariant?feature?transform (SIFT) descriptor binaryzation and similarity matching method
A similarity matching and binarization technology, applied in the field of image matching, can solve problems such as the huge amount of face image data, and achieve the effects of simple and fast calculation, reduced complexity, and good real-time performance.
- Summary
- Abstract
- Description
- Claims
- Application Information
AI Technical Summary
Problems solved by technology
Method used
Image
Examples
Embodiment Construction
[0031] The overall process of the technical solution of the present invention is as follows in the description attached figure 1shown. Our method greatly reduces the amount of data storage, reduces the complexity of calculation, and can better meet the requirements of real-time performance. And the matching result equivalent to the original SIFT descriptor can be obtained, which is far better than the result of uniform binarization of the SIFT descriptor for matching.
[0032] A. For each face image, first extract the SIFT feature descriptor, and then binarize the SIFT descriptor to obtain the information entropy of each level, according to the probability of occurrence of 0 and 1 in each layer and the total number of bits reserved , to obtain the bit average entropy, and then find out the number of binarization layers corresponding to the maximum bit average entropy, keep the binarization results of these layers, and finally form a new binarization descriptor. Specific step...
PUM
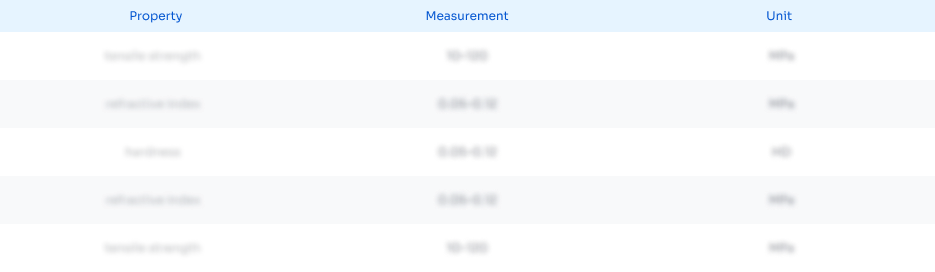
Abstract
Description
Claims
Application Information
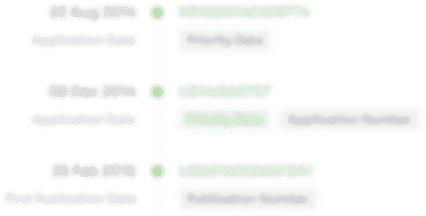
- R&D Engineer
- R&D Manager
- IP Professional
- Industry Leading Data Capabilities
- Powerful AI technology
- Patent DNA Extraction
Browse by: Latest US Patents, China's latest patents, Technical Efficacy Thesaurus, Application Domain, Technology Topic, Popular Technical Reports.
© 2024 PatSnap. All rights reserved.Legal|Privacy policy|Modern Slavery Act Transparency Statement|Sitemap|About US| Contact US: help@patsnap.com