Electrocardiogram electrocardiosignal classification method with multi-scale characteristics combined
A multi-scale feature, ECG signal technology, applied in the multi-scale feature fusion, the classification of normal and various abnormal ECG signals, can solve the signal feature redundancy, resolution is not enough, can not be well expressed Signal and other problems to achieve the effect of improving classification accuracy and reducing classification time
- Summary
- Abstract
- Description
- Claims
- Application Information
AI Technical Summary
Problems solved by technology
Method used
Image
Examples
Embodiment Construction
[0018] The rapid multi-scale feature fusion technology method provided by the present invention to improve the heartbeat signal classification accuracy will be described in detail below in conjunction with the accompanying drawings.
[0019] figure 1 It is a flow chart of the heartbeat signal classification method, including the following steps:
[0020] Step S101, read all ECG signals in the database.
[0021] Step S102, removing the baseline and high-frequency noise of the signal.
[0022] (1) Apply median filters with bandwidths of 200ms and 600ms to remove ORS complexes and P&T waves respectively, and subtract them from the original signal to obtain the baseline-removed signal.
[0023] (2) Apply a low-pass filter to remove high-frequency noise in the signal.
[0024] Step S103, segmenting the ECG signal: first find the reference point of the ECG signal (that is, point R), and use 99 sampling points forward and 100 sampling points backward from the reference point as a ...
PUM
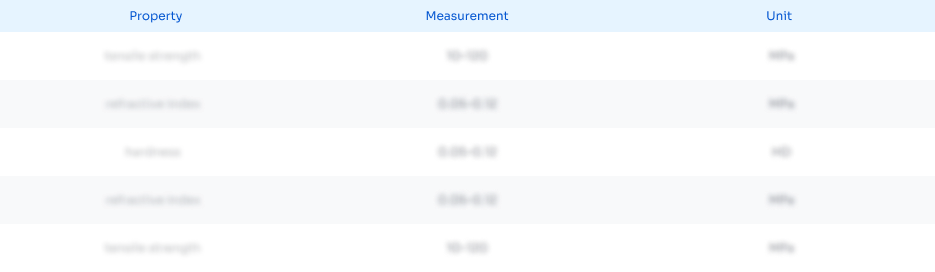
Abstract
Description
Claims
Application Information
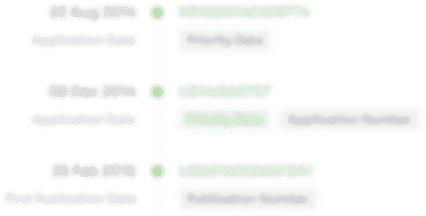
- R&D Engineer
- R&D Manager
- IP Professional
- Industry Leading Data Capabilities
- Powerful AI technology
- Patent DNA Extraction
Browse by: Latest US Patents, China's latest patents, Technical Efficacy Thesaurus, Application Domain, Technology Topic, Popular Technical Reports.
© 2024 PatSnap. All rights reserved.Legal|Privacy policy|Modern Slavery Act Transparency Statement|Sitemap|About US| Contact US: help@patsnap.com