Blind source signal denoising method based on ensemble empirical mode decomposition
A technology that integrates empirical modes and blind source signals. It is applied in the field of signal processing and can solve the problems of Newton's method not converging and objective function value not converging.
- Summary
- Abstract
- Description
- Claims
- Application Information
AI Technical Summary
Problems solved by technology
Method used
Image
Examples
Embodiment 1
[0061] In order to prove the effectiveness and superiority of the IEEMD method in processing speech signals, a test speech is used for specific analysis below. The selected voice signal is a section of female audio in the TIMIT voice library, and the content is: "She had your dark suit in greasy wash water all year".
[0062] The present invention is based on the blind source signal denoising method of ensemble empirical mode decomposition: firstly, the original EEMD algorithm is revised; secondly, the N-order IMF obtained by decomposing the revised EEMD is analyzed through stepwise regression, and false components are eliminated; then all IMFs are passed through ICA performs signal separation and reconstruction to maximize signal enhancement and eliminate noise; specifically includes the following steps: specifically includes the following steps:
[0063] First, through the enumeration experiment, that is, the signal is decomposed in the case of multiple groups of different w...
Embodiment 2
[0112] The difference from Example 1 is that this example selects a section of female audio in the TIMIT voice database as the experimental corpus for the analysis of the actual voice signal. The original sampling frequency of the voice signal is 16kHz, monophonic recording, 16bit quantization, the content is: "She had your dark suit in greasy wash water all year". figure 2 It is the 15th-order IMF component obtained after IEEMD decomposition. Unlike the simulation signal, it is impossible to intuitively judge which IMF component is a false component after the speech signal is decomposed by IEEMD, and the contribution of each IMF component to the original voice cannot be determined. Intuitive judgment.
[0113] After stepwise regression analysis of the 15th-order IMF components obtained by IEEMD decomposition, the results are shown in Table 1:
[0114] Table 1 IMF regression coefficients
[0115]
[0116] It can be clearly seen from Table 1 that the components after IMF8...
Embodiment 3
[0119] Different from Embodiment 1, this example defines the noise-added speech signal as y(t)=s(t)+n(t), where s(t) is a pure speech signal and n(t) is a noise signal. In order to visually verify the effectiveness of the algorithm proposed in this paper, a piece of female audio and a male audio were randomly selected in the TIMIT speech library for analysis, and the added noise signal n(t) is the Vehicle interior noise, F in the standard noise library noisex-92 -16 noise and factory noise. These three noises are added to the pure speech according to the signal-to-noise ratios of -5dB, -10dB, and -15dB. The input signal-to-noise ratio and output signal-to-noise ratio are defined as formulas (17) and (18).
[0120] SNR IN = 10 log 10 Σ i = 1 n ...
PUM
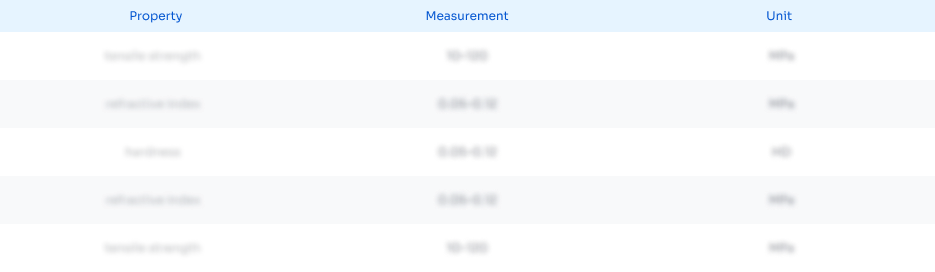
Abstract
Description
Claims
Application Information
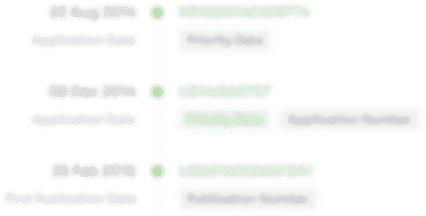
- R&D Engineer
- R&D Manager
- IP Professional
- Industry Leading Data Capabilities
- Powerful AI technology
- Patent DNA Extraction
Browse by: Latest US Patents, China's latest patents, Technical Efficacy Thesaurus, Application Domain, Technology Topic.
© 2024 PatSnap. All rights reserved.Legal|Privacy policy|Modern Slavery Act Transparency Statement|Sitemap