Electrocardiogram classifying method based on fuzzy inference and weighted similarity measurement
A technology of similarity measurement and fuzzy reasoning, applied in the direction of reasoning methods, electrical digital data processing, special data processing applications, etc., can solve the problems of high classification error rate, failure to build ECG knowledge base, etc., to narrow the matching range and solve the problem of construction problem, the effect of reducing the probability of misclassification
- Summary
- Abstract
- Description
- Claims
- Application Information
AI Technical Summary
Problems solved by technology
Method used
Image
Examples
specific Embodiment approach 1
[0020] Specific implementation mode one: combine figure 1 Illustrate this embodiment, a kind of ECG classification method based on fuzzy reasoning combined with weighted similarity measure, comprises the following steps:
[0021] Step 1: Preprocessing the known type of ECG signal f(n), including two parts, using the mathematical morphology method to remove the baseline drift, using the wavelet threshold method to remove high-frequency noise, and using the preprocessed ECG signal with F (n) means;
[0022] figure 2 The processing results of removing baseline drift are given, where figure 2 (a) represents the original ECG signal, figure 2 (b) represents the ECG signal after removing the baseline drift. image 3 The processing results of removing high-frequency noise are given, where image 3 (a) represents the original ECG signal, image 3 (b) represents the ECG signal after removing the baseline drift.
[0023] Step 2: Carry out waveform detection to the ECG signal F(...
specific Embodiment approach 2
[0030]Specific embodiment two: in step two described in the present embodiment, carry out the waveform detection of QRS band to the electrocardiogram signal F (n) of known type and the realization process of extracting corresponding feature parameter is:
[0031] Step 2.1.1, utilize binary spline wavelet transform to decompose and filter electrocardiographic signal F (n) by Mallat algorithm, eliminate interference;
[0032] Step 2.1.2, using the relationship between wavelet transform and signal singularity, in 2 3 The QRS band is detected under the scale to obtain the start and end points of the QRS band. If the number of detected sampling points in the QRS band is greater than 36, it is judged that the QRS band is wide, and if it is less than or equal to 36, it is judged that the QRS band is normal;
[0033] Step 2.1.3, obtain the number of heart beats with wide QRS bands in all ECG signals F(n) in the known type i, and count the number of heart beats with wide QRS bands, and...
specific Embodiment approach 3
[0036] Specific embodiment three: in step 2 of this embodiment, the implementation process of performing T wave waveform detection and extracting corresponding characteristic parameters to the known type of electrocardiographic signal F(n) is:
[0037] Step 2.2.1, utilize the method of wavelet extremum point to detect T wave, obtain the starting and ending point of T wave; By analyzing wavelet transform in 2 1 -2 5 The wavelet coefficients on the scale can be known: scale 2 4 and 2 5 contains most of the energy of the T wave, but scale 2 5 Contains part of the baseline drift noise; so 2 4 scale wavelet transform, looking for extreme points on this scale, and searching for the positive modulus maximum value P with the largest amplitude between the start and end points of the T wave max and negative modulus maximum P min , set the amplitude thresholds of the positive and negative modulus maxima to be P max / 6 and P min / 6, satisfying P i max / 6 and P i >P min The modul...
PUM
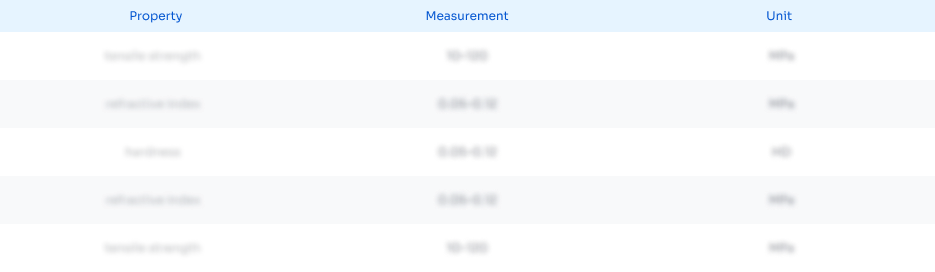
Abstract
Description
Claims
Application Information
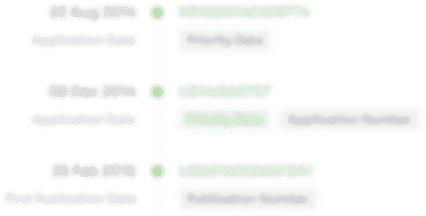
- R&D Engineer
- R&D Manager
- IP Professional
- Industry Leading Data Capabilities
- Powerful AI technology
- Patent DNA Extraction
Browse by: Latest US Patents, China's latest patents, Technical Efficacy Thesaurus, Application Domain, Technology Topic, Popular Technical Reports.
© 2024 PatSnap. All rights reserved.Legal|Privacy policy|Modern Slavery Act Transparency Statement|Sitemap|About US| Contact US: help@patsnap.com