Image classification and searching method based on geographic position characteristics and overall situation vision characteristics
A technology of visual features and geographic location, applied in still image data retrieval, still image data indexing, electrical digital data processing, etc., can solve the problems of time-consuming, low precision, unable to guarantee user location service requirements, etc., and achieve retrieval efficiency. Improves, reduces time-consuming effects
- Summary
- Abstract
- Description
- Claims
- Application Information
AI Technical Summary
Problems solved by technology
Method used
Image
Examples
specific Embodiment approach 1
[0024] Specific implementation mode one: combine figure 1 , figure 2 and image 3 To illustrate this embodiment, the image classification and retrieval method based on geographic location features and global visual features is specifically carried out in accordance with the following steps:
[0025] Step 1. Database initialization:
[0026] Collect images with geographic location information outdoors and store them as database data;
[0027] Step 2. Database image preprocessing:
[0028] Convert the image in the database into a grayscale image, extract the Gist feature as a global visual feature, and express it with a vector G, then use the position information of the grayscale image in the database as the position feature L of the grayscale image, and the fusion feature of the grayscale image in the database vector for F t ={αG,(1-α)L};
[0029] Step 3, database image clustering:
[0030] Utilize the K-means algorithm to cluster the fusion feature vector in step 2, an...
specific Embodiment approach 2
[0035] Specific embodiment 2: The difference between this embodiment and specific embodiment 1 is: the database image preprocessing in the step 2: convert the image in the database into a grayscale image, extract the Gist feature as the global visual feature, and represent it with a vector G, Then the location information of the grayscale image in the database is used as the location feature L of the grayscale image, and the fusion feature vector of the grayscale image in the database is F t ={αG,(1-α)L}; the specific process is:
[0036] (1) Scale the size of the image in the database to 300*300 pixels in proportion, and convert it into a grayscale image. The grayscale value of the grayscale image is an integer in the range of 0 to 255;
[0037] Divide the grayscale image into a 3*3 regular grid, the number of grid blocks is 9, and each grid pixel is 100*100 pixels;
[0038] Divide the gray value range into 8 scales equidistantly, the gray value range of scale 1 is 0-31, the...
specific Embodiment approach 3
[0057] Specific embodiment three: the difference between this embodiment and specific embodiment one or two is: the database image clustering in the step three: use the K-means algorithm to cluster the fusion feature vector in the step two, and calculate the clustering Center, after obtaining the clustering center, use the global visual feature vector in the vector as the visual feature of the grayscale image category in the database; the specific process is:
[0058] Step 31, randomly select p grayscale images from the database, and use the fusion feature vectors of these grayscale images as initial clustering centers;
[0059] Step 32. Calculate the Euclidean distance between the fusion feature vector of each grayscale image in the database and each initial cluster center, as shown in formula (6):
[0060] d ( F 1 , F 2 ) ...
PUM
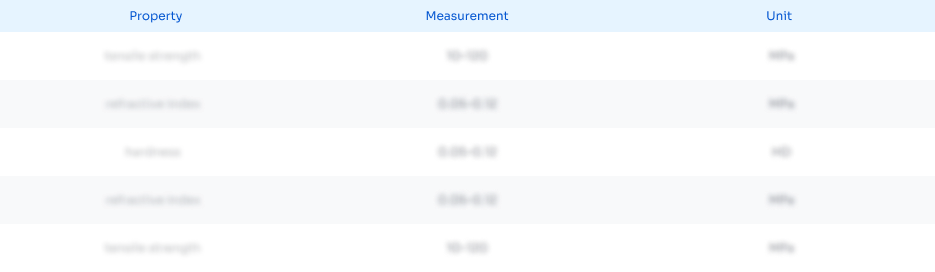
Abstract
Description
Claims
Application Information
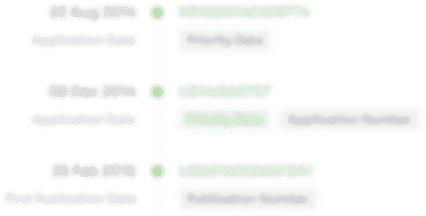
- R&D Engineer
- R&D Manager
- IP Professional
- Industry Leading Data Capabilities
- Powerful AI technology
- Patent DNA Extraction
Browse by: Latest US Patents, China's latest patents, Technical Efficacy Thesaurus, Application Domain, Technology Topic, Popular Technical Reports.
© 2024 PatSnap. All rights reserved.Legal|Privacy policy|Modern Slavery Act Transparency Statement|Sitemap|About US| Contact US: help@patsnap.com