Compressed sensing video reconstruction method based on dictionary learning residual-error reconstruction
A technology of compressed sensing and dictionary learning, applied in digital video signal modification, electrical components, image communication, etc., can solve problems such as poor image quality, poor quality, and poor adaptability, and achieve enhanced sparsity, improved fidelity, and increased The effect of high compression
- Summary
- Abstract
- Description
- Claims
- Application Information
AI Technical Summary
Problems solved by technology
Method used
Image
Examples
Embodiment Construction
[0043] The principles and features of the present invention are described below in conjunction with the accompanying drawings, and the examples given are only used to explain the present invention, and are not intended to limit the scope of the present invention.
[0044] Such as figure 1 Shown is the general flow chart of the present invention relating to a compressed sensing video reconstruction method based on dictionary learning residual reconstruction; figure 2 It is a flow chart of the steps of video coding based on compressed sensing involved in the invention; image 3 It is a flow chart of the steps involved in the present invention to obtain the initial reconstruction of GOP; Figure 4 A flow chart of the steps involved in the present invention to obtain the final reconstruction of the GOP; Figure 5 It is a flow chart of the steps of the method for obtaining motion compensation using multiple reference frames involved in the present invention; Figure 6 It is a f...
PUM
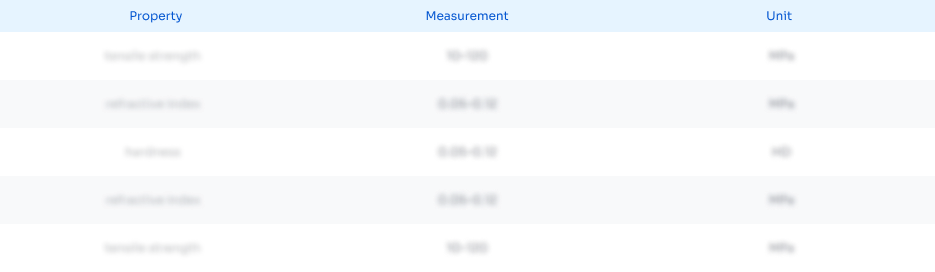
Abstract
Description
Claims
Application Information
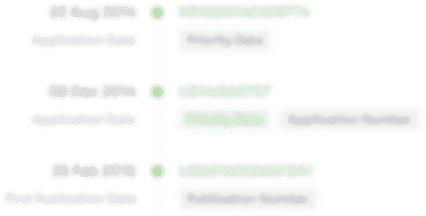
- R&D Engineer
- R&D Manager
- IP Professional
- Industry Leading Data Capabilities
- Powerful AI technology
- Patent DNA Extraction
Browse by: Latest US Patents, China's latest patents, Technical Efficacy Thesaurus, Application Domain, Technology Topic.
© 2024 PatSnap. All rights reserved.Legal|Privacy policy|Modern Slavery Act Transparency Statement|Sitemap