SAR Image Classification Method Based on Hierarchical Sparse Filter Convolutional Neural Network
A convolutional neural network and sparse filtering technology, which is applied in the field of SAR image classification and target recognition, can solve problems such as limited application, low classification accuracy, and difficult to overcome the influence of noise, and achieve overcoming coherent speckle noise, high classification accuracy, and stable The effect of classification results
- Summary
- Abstract
- Description
- Claims
- Application Information
AI Technical Summary
Problems solved by technology
Method used
Image
Examples
Embodiment Construction
[0032] refer to figure 1 , the realization steps of the present invention are as follows.
[0033] Step 1: Divide the SAR image database sample set into training data set x and test sample set y.
[0034] Firstly, 1000 pictures with a size of 256×256 are taken in each sample set including 6 types of SAR image database sample sets, and then 200 pictures are randomly selected from each type of pictures to form a training set x, and the rest are used as a test set y.
[0035] Step 2: randomly extract m training image blocks of size d×d from the training data set x, and perform global contrast normalization to form a training image block set
[0036] Step 3: Use the training image patch set X to train the first layer of sparse dictionary.
[0037] 3a) Express the feature matrix of the training image block set X as:
[0038]
[0039] in Represents a dictionary, N represents the number of features of each image block, ε is a very small constant, F∈R m×N Represents the fe...
PUM
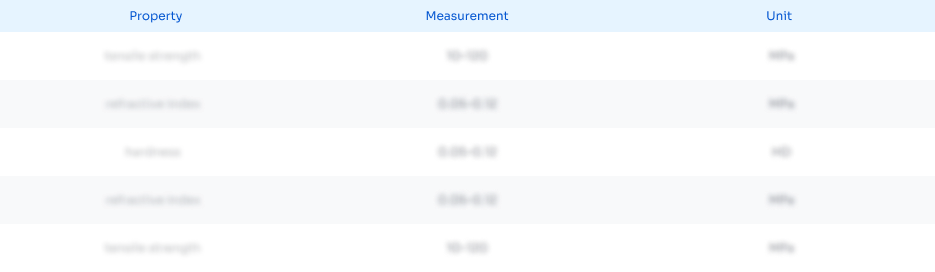
Abstract
Description
Claims
Application Information
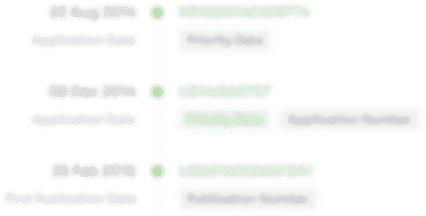
- R&D Engineer
- R&D Manager
- IP Professional
- Industry Leading Data Capabilities
- Powerful AI technology
- Patent DNA Extraction
Browse by: Latest US Patents, China's latest patents, Technical Efficacy Thesaurus, Application Domain, Technology Topic, Popular Technical Reports.
© 2024 PatSnap. All rights reserved.Legal|Privacy policy|Modern Slavery Act Transparency Statement|Sitemap|About US| Contact US: help@patsnap.com