Fatigue Driving Evaluation Method Based on Artificial Neural Network and Evidence Theory
A technology of artificial neural network and evidence theory, applied in the direction of biological neural network model, etc., can solve problems such as difficult detection and evaluation, unsatisfactory evaluation effect, abnormal vehicle driving state, etc.
- Summary
- Abstract
- Description
- Claims
- Application Information
AI Technical Summary
Problems solved by technology
Method used
Image
Examples
Embodiment Construction
[0136] The present invention will be further described below in conjunction with the accompanying drawings. The following examples are only used to illustrate the technical solution of the present invention more clearly, but not to limit the protection scope of the present invention.
[0137] Such as figure 1 As shown, the fatigue driving evaluation method based on artificial neural network and evidence theory includes the following steps:
[0138] Step 1, collecting fatigue feature data samples for artificial neural network training.
[0139] The sample collection process of fatigue characteristic data is as follows:
[0140] A1) Recruit several test drivers, and conduct self-evaluation according to fatigue state.
[0141] The recruited test drivers generally need to have at least 3 years of driving experience, be in good health, have no disease, be in a good mood, and have not drunk alcohol or strong tea or coffee 24 hours before the experiment. Before the test, the test...
PUM
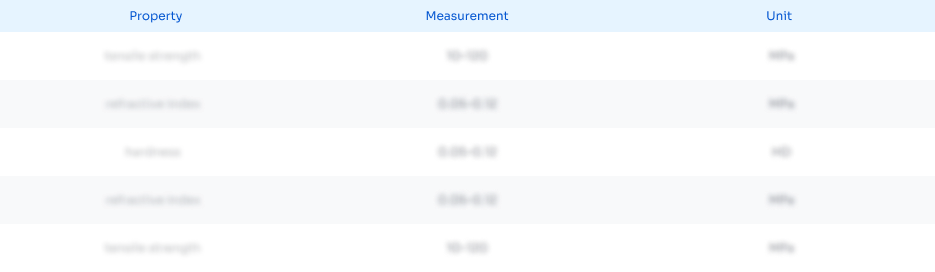
Abstract
Description
Claims
Application Information
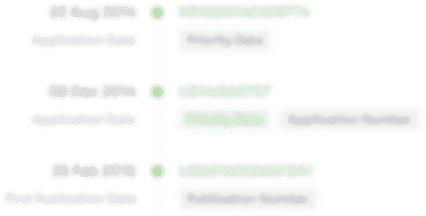
- R&D
- Intellectual Property
- Life Sciences
- Materials
- Tech Scout
- Unparalleled Data Quality
- Higher Quality Content
- 60% Fewer Hallucinations
Browse by: Latest US Patents, China's latest patents, Technical Efficacy Thesaurus, Application Domain, Technology Topic, Popular Technical Reports.
© 2025 PatSnap. All rights reserved.Legal|Privacy policy|Modern Slavery Act Transparency Statement|Sitemap|About US| Contact US: help@patsnap.com