Deep neural network system based on memristor
A deep neural network and memristive device technology, applied in the field of deep neural network systems, can solve problems such as high overhead and difficult application in embedded fields, and achieve the effect of increasing computing speed and density and reducing operating energy consumption
- Summary
- Abstract
- Description
- Claims
- Application Information
AI Technical Summary
Problems solved by technology
Method used
Image
Examples
Embodiment Construction
[0016] The deep neural network system based on the memristive device provided by the present invention will be further described in detail below with reference to the drawings and specific embodiments.
[0017] See figure 2 An embodiment of the present invention provides a deep neural network system 10 based on a memristive device, including: an input layer 11 , a plurality of hidden layers 12 and an output layer 13 . The input layer 11 is connected to the plurality of hidden layers 12 , and the input layer 11 receives an external information input pattern 14 and sends the input pattern 14 to the plurality of hidden layers 12 . The multiple hidden layers 12 are respectively connected with the input layer 11 and the output layer 13, and the multiple hidden layers 12 perform layer-by-layer calculation conversion on the input pattern 14 from the input layer 11, and send the calculation results to The output layer 13 . The output layer 13 receives the calculation result of the ...
PUM
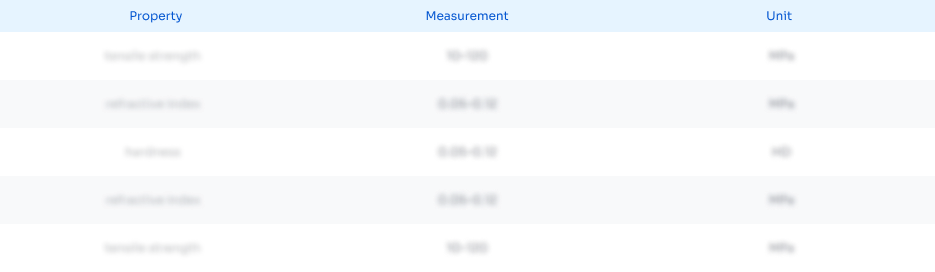
Abstract
Description
Claims
Application Information
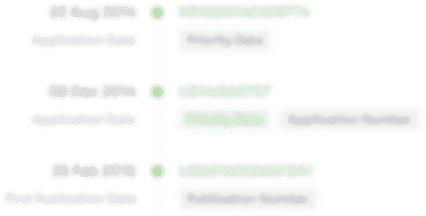
- R&D Engineer
- R&D Manager
- IP Professional
- Industry Leading Data Capabilities
- Powerful AI technology
- Patent DNA Extraction
Browse by: Latest US Patents, China's latest patents, Technical Efficacy Thesaurus, Application Domain, Technology Topic, Popular Technical Reports.
© 2024 PatSnap. All rights reserved.Legal|Privacy policy|Modern Slavery Act Transparency Statement|Sitemap|About US| Contact US: help@patsnap.com