Method for dynamic prediction of air-conditioning loads based on multi-factor chaos support vector machine
A support vector machine and air-conditioning load technology, applied in forecasting, chaos model, information technology support system, etc., can solve the problems of not fully considering the internal causes of air-conditioning load, heavy forecasting workload, lack of scientific guidance, etc., and achieve modeling work The effect of small amount, simple acquisition, and reasonable selection of training data
- Summary
- Abstract
- Description
- Claims
- Application Information
AI Technical Summary
Problems solved by technology
Method used
Image
Examples
Embodiment Construction
[0018] The present invention will be described in further detail below in conjunction with the accompanying drawings and specific embodiments.
[0019] Such as figure 1 The above is the schematic diagram of the multi-factor chaos support vector machine load forecasting model. The multi-factor chaos support vector machine load forecasting model, the input parameters are the load time series after phase space reconstruction, outdoor temperature, relative humidity, solar radiation intensity, and chilled water temperature and time. The input parameter is the hourly load for the second day. The formation of the support vector machine decision function is similar to a neural network. The output is a linear combination of intermediate nodes. Each intermediate node corresponds to the inner product of a prediction sample and a support vector. The number of intermediate nodes is the number L of support vectors.
[0020] 1) Establish a training sample set: For the existing multi-factor...
PUM
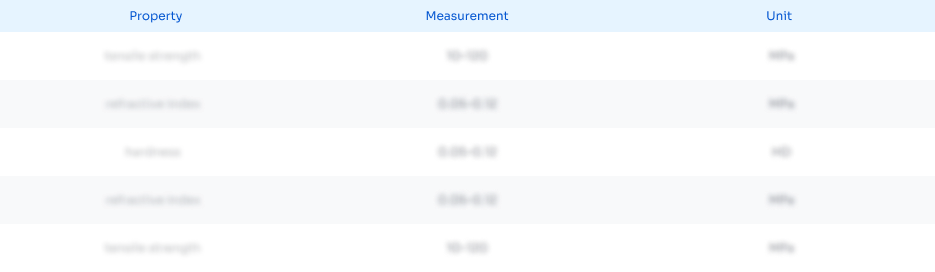
Abstract
Description
Claims
Application Information
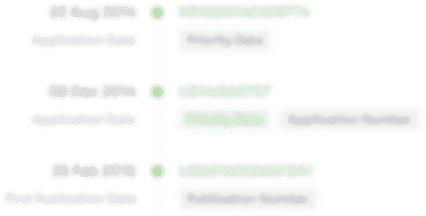
- R&D Engineer
- R&D Manager
- IP Professional
- Industry Leading Data Capabilities
- Powerful AI technology
- Patent DNA Extraction
Browse by: Latest US Patents, China's latest patents, Technical Efficacy Thesaurus, Application Domain, Technology Topic, Popular Technical Reports.
© 2024 PatSnap. All rights reserved.Legal|Privacy policy|Modern Slavery Act Transparency Statement|Sitemap|About US| Contact US: help@patsnap.com