Joint embedded model for zero sample learning
A sample learning and model technology, applied in computer parts, character and pattern recognition, instruments, etc., can solve problems such as high complexity, long training time, and difficulty in association, and achieve the effect of low complexity
- Summary
- Abstract
- Description
- Claims
- Application Information
AI Technical Summary
Problems solved by technology
Method used
Image
Examples
Embodiment Construction
[0020] A joint embedding model for zero-shot learning of the present invention will be described in detail below with reference to embodiments and drawings.
[0021] figure 1 The main flow of a joint embedding model for zero-shot learning of the present invention is described. In the training phase, first extract features from the image and text, extract visual features from the image and use the language model to extract the text vector corresponding to the image from the corpus, and then use the algorithm provided by the present invention to learn to be able to associate different modal features The transformation matrix; in the test phase, first extract the image visual features of unseen categories, and then use the learned feature transformation matrix to transform the visual features into feature descriptions in the text feature space, and correspond to the text features closest to the transformed features The category of is used as the category of the test image.
[0...
PUM
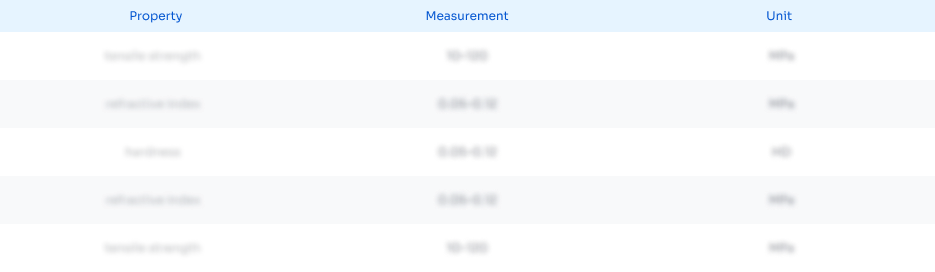
Abstract
Description
Claims
Application Information
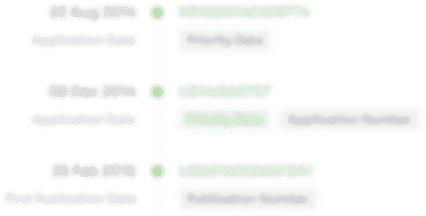
- R&D
- Intellectual Property
- Life Sciences
- Materials
- Tech Scout
- Unparalleled Data Quality
- Higher Quality Content
- 60% Fewer Hallucinations
Browse by: Latest US Patents, China's latest patents, Technical Efficacy Thesaurus, Application Domain, Technology Topic, Popular Technical Reports.
© 2025 PatSnap. All rights reserved.Legal|Privacy policy|Modern Slavery Act Transparency Statement|Sitemap|About US| Contact US: help@patsnap.com