Real-time limit learning machine short-time traffic flow prediction method based on fusion
A technology of short-term traffic flow and extreme learning machine, which is applied in the field of fusion-based real-time extreme learning machine short-term traffic flow prediction. , uneven distribution of heterogeneity value data, etc.
- Summary
- Abstract
- Description
- Claims
- Application Information
AI Technical Summary
Problems solved by technology
Method used
Image
Examples
Embodiment Construction
[0070] The present invention will be further described below in conjunction with the accompanying drawings and specific preferred embodiments, but the protection scope of the present invention is not limited thereby.
[0071] refer to figure 1 , figure 2 As shown, the fusion-based real-time sequence extreme learning machine short-term traffic flow prediction method mainly includes the following steps:
[0072] Step 1. Collect short-term traffic flow data. The present invention uses the PeMS system to randomly select the detection points of four highways in California, USA to obtain the traffic flow historical data and perform prediction analysis. And randomly select the traffic flow data from 2014-11-24 to 2014-12-1, the data includes the traffic flow data of normal working days and holidays, which can represent the scenes of smooth and non-stationary periods. Among them, the data of the first 7 days is used as the training set, and the data of the last day is used as the ...
PUM
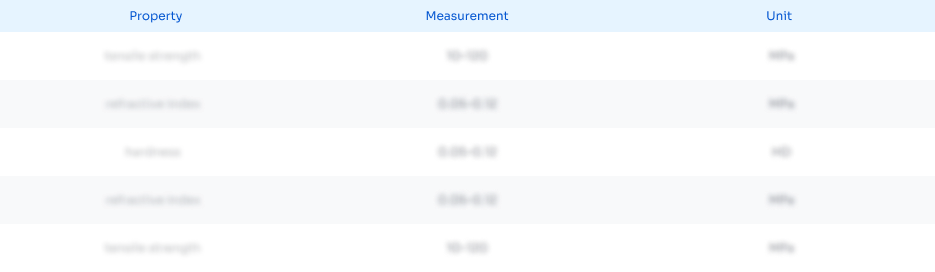
Abstract
Description
Claims
Application Information
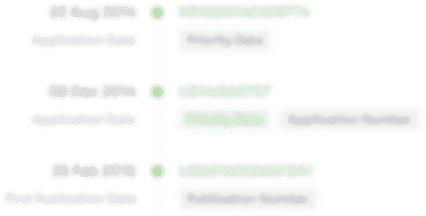
- R&D
- Intellectual Property
- Life Sciences
- Materials
- Tech Scout
- Unparalleled Data Quality
- Higher Quality Content
- 60% Fewer Hallucinations
Browse by: Latest US Patents, China's latest patents, Technical Efficacy Thesaurus, Application Domain, Technology Topic, Popular Technical Reports.
© 2025 PatSnap. All rights reserved.Legal|Privacy policy|Modern Slavery Act Transparency Statement|Sitemap|About US| Contact US: help@patsnap.com