Distribution method oriented to simulation Q learning attack targets
A target allocation and learning algorithm technology, applied in the field of combat simulation, can solve the problems of poor local search ability, prone to premature phenomenon, and easy to fall into the trap of local optimality.
- Summary
- Abstract
- Description
- Claims
- Application Information
AI Technical Summary
Problems solved by technology
Method used
Image
Examples
Embodiment Construction
[0018] The present invention will be described in detail below in conjunction with the accompanying drawings.
[0019] Attack target allocation in air combat refers to formulating combat intentions based on combat missions and theater air combat situation monitoring, dispatching and controlling the aircraft resources of the entire fleet in units of fighter formations, and assigning corresponding formations. The concept is as follows: figure 1 shown. The invention proposes a simulation-oriented Q-learning attack target allocation method, which is used to obtain the optimization result of red fighter formation target allocation in air combat simulation.
[0020] The steps of the present invention include:
[0021] (1) Determine the initial state, obtain the air combat situation information of both sides, including the number of aircraft in the formation of both sides and the parameters related to the formation aircraft, and provide input for the red team target allocation and a...
PUM
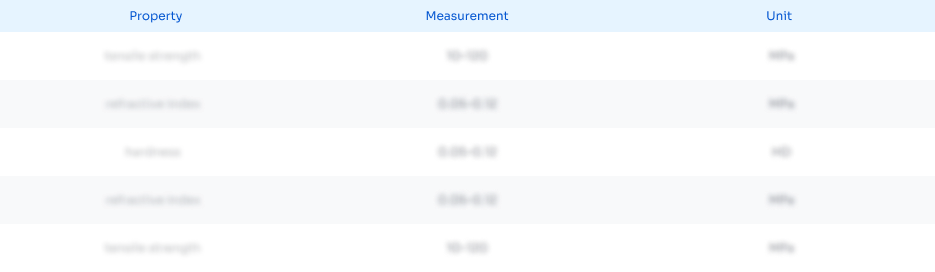
Abstract
Description
Claims
Application Information
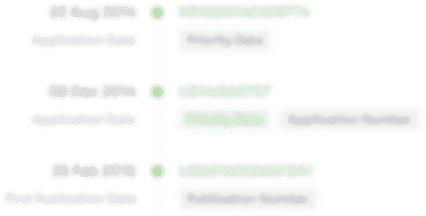
- Generate Ideas
- Intellectual Property
- Life Sciences
- Materials
- Tech Scout
- Unparalleled Data Quality
- Higher Quality Content
- 60% Fewer Hallucinations
Browse by: Latest US Patents, China's latest patents, Technical Efficacy Thesaurus, Application Domain, Technology Topic, Popular Technical Reports.
© 2025 PatSnap. All rights reserved.Legal|Privacy policy|Modern Slavery Act Transparency Statement|Sitemap|About US| Contact US: help@patsnap.com