Deep-learning-based partial discharge defective image diagnosing method and system
A technology of partial discharge and deep learning, applied in the direction of optical testing flaws/defects, using optical methods for testing, scientific instruments, etc., can solve problems such as difficult to solve complex classification, low accuracy of fault diagnosis and recognition, and achieve the goal of improving accuracy Effect
- Summary
- Abstract
- Description
- Claims
- Application Information
AI Technical Summary
Problems solved by technology
Method used
Image
Examples
Embodiment Construction
[0047] The present invention will be described in detail below in conjunction with specific embodiments. The following examples will help those skilled in the art to further understand the present invention, but do not limit the present invention in any form. It should be noted that those skilled in the art can make several changes and improvements without departing from the concept of the present invention. These all belong to the protection scope of the present invention.
[0048] The partial discharge defect image diagnosis method based on deep learning provided by the present invention comprises the following steps:
[0049] Step 1: Detect the partial discharge signal of the power equipment, and obtain the partial discharge defect image;
[0050] Step 2: Establish a partial discharge defect image sample library, extract training set and test set;
[0051] Step 3: Establish a deep convolutional neural network model, use samples for deep learning training and testing, and...
PUM
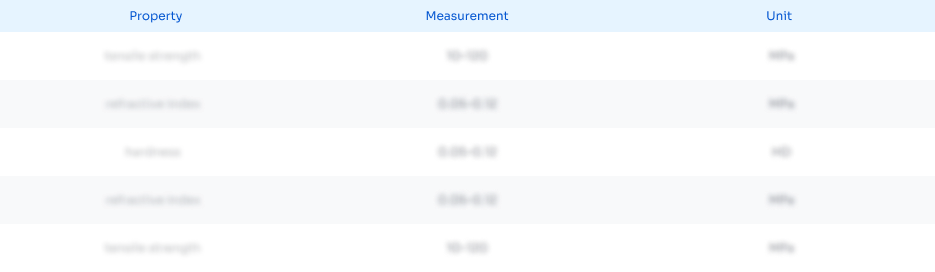
Abstract
Description
Claims
Application Information
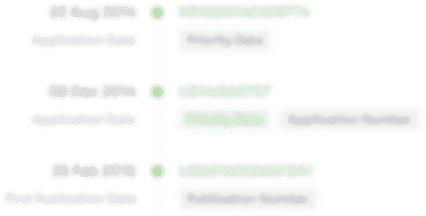
- R&D Engineer
- R&D Manager
- IP Professional
- Industry Leading Data Capabilities
- Powerful AI technology
- Patent DNA Extraction
Browse by: Latest US Patents, China's latest patents, Technical Efficacy Thesaurus, Application Domain, Technology Topic, Popular Technical Reports.
© 2024 PatSnap. All rights reserved.Legal|Privacy policy|Modern Slavery Act Transparency Statement|Sitemap|About US| Contact US: help@patsnap.com