Object-level depth feature aggregation method for image retrieval
A deep feature, image retrieval technology, applied in special data processing applications, instruments, electrical digital data processing and other directions, can solve the problems of redundancy, lost information, noise and other problems in the aggregation process, to reduce complexity, improve accuracy, high The effect of robustness
- Summary
- Abstract
- Description
- Claims
- Application Information
AI Technical Summary
Problems solved by technology
Method used
Image
Examples
Embodiment 1
[0023] Example 1: Retrieval of similar images
[0024] 1. figure 1 It is a flow chart of the present invention. Firstly, all images in the library image are extracted using the fast mode of the Selective Search algorithm to extract candidate areas. On average, about 2000 candidate areas of different sizes can be obtained for each image.
[0025] 2. The present invention adopts the convolutional neural network structure Alex network of Krizhevsky et al. The input is an RGB image of 224*224, including five layers of convolution layers, three layers of maximum pooling layers and three layers of fully connected layers. The network is trained using the Caffe framework, and the training data is the 1000-class classification data set in the ILSVRC12 competition.
[0026] 3. After the network training is completed, the candidate area obtained in step 1 is filled and scaled to a fixed size of 224*224 as the input of the network, and the output of the fully connected layer fc7 is extra...
PUM
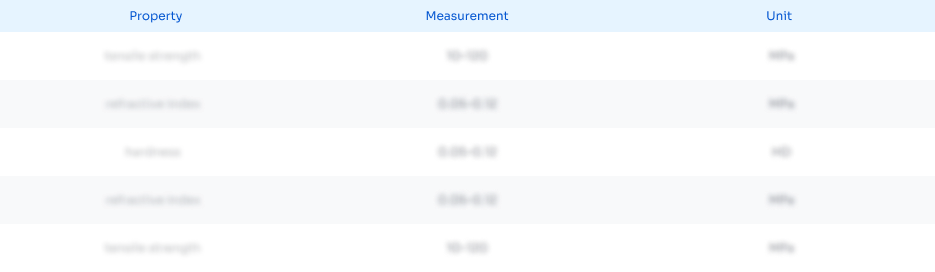
Abstract
Description
Claims
Application Information
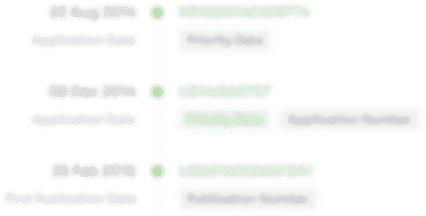
- R&D
- Intellectual Property
- Life Sciences
- Materials
- Tech Scout
- Unparalleled Data Quality
- Higher Quality Content
- 60% Fewer Hallucinations
Browse by: Latest US Patents, China's latest patents, Technical Efficacy Thesaurus, Application Domain, Technology Topic, Popular Technical Reports.
© 2025 PatSnap. All rights reserved.Legal|Privacy policy|Modern Slavery Act Transparency Statement|Sitemap|About US| Contact US: help@patsnap.com