Factory bus load prediction based on similar day and least squares support vector machine
A technology of support vector machine and bus load, which is used in forecasting, instrumentation, data processing applications, etc., and can solve the problems of difficult bus load forecasting, sudden changes in data, and low forecast accuracy.
- Summary
- Abstract
- Description
- Claims
- Application Information
AI Technical Summary
Problems solved by technology
Method used
Image
Examples
Embodiment 1
[0070] (1) Select the data sample of 35kV busbar load dedicated to a large metallurgical enterprise in May (31 days). The sample includes the maximum temperature, minimum temperature, average temperature, relative humidity, date type (weekday, weekend) and load data ( 24 points per day); taking the 31st as the forecast day, using SOM’s fuzzy clustering model and artificial experience to select ten similar days from the data of the other 30 days, the results are shown in Table 1;
[0071] Table 1 Similar days selected by artificial and SOM fuzzy clustering methods
[0072]
[0073] The 10 similar days selected by the two methods were used as training samples, and May 31 was used as the prediction day, and the least squares support vector machine model was used for prediction. The results are shown in Table 2;
[0074] Table 2 Artificial and SOM fuzzy clustering methods select similar days for
[0075]
[0076] It can be seen from Table 2 that using the SOM fuzzy clusteri...
Embodiment 2
[0095] Here, the 30th, 29th, and 28th of June are the forecast days. Select ten similar days, after wavelet denoising preprocessing, and then use chaotic particle swarm optimization to optimize the least squares support vector machine model prediction; the predicted load value is as follows Figure 5 Shown, MRE value, RMSE value and A L Values are shown in Table 6;
[0096] Table 6 Optimizing the least squares support vector machine with chaotic particle swarm optimization on bus 2
[0097] The model continuously predicts the MRE value, RMSE value and A L value
[0098]
[0099] From attached Figure 5 It can be seen from Table 6 that in the 72 time points of continuous prediction for 3 days, the MRE value is less than 2%, and A L The value is greater than 97%, indicating that the load prediction of bus 2 by the least squares support vector machine model optimized by chaotic particle swarm optimization meets the prediction accuracy requirements.
PUM
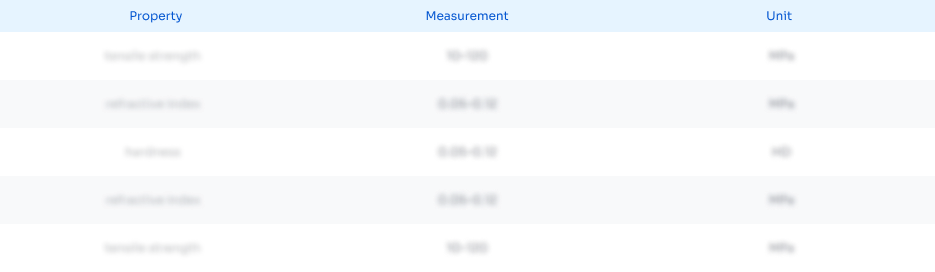
Abstract
Description
Claims
Application Information
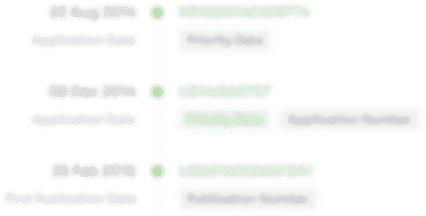
- R&D Engineer
- R&D Manager
- IP Professional
- Industry Leading Data Capabilities
- Powerful AI technology
- Patent DNA Extraction
Browse by: Latest US Patents, China's latest patents, Technical Efficacy Thesaurus, Application Domain, Technology Topic, Popular Technical Reports.
© 2024 PatSnap. All rights reserved.Legal|Privacy policy|Modern Slavery Act Transparency Statement|Sitemap|About US| Contact US: help@patsnap.com