Sewage treatment fault diagnosis method based on weighted extreme learning machine integrated algorithm
An extreme learning machine and fault diagnosis technology, applied in computing, computer parts, instruments, etc., can solve problems such as uneven distribution of sewage data sets, substandard effluent quality, and difficulty in sewage treatment plants, and solve the problem of data distribution between classes Unbalanced problem, imbalanced distribution between good data classes, and the effect of speeding up classification learning
- Summary
- Abstract
- Description
- Claims
- Application Information
AI Technical Summary
Problems solved by technology
Method used
Image
Examples
Example Embodiment
[0044] Example
[0045] See attached figure 1 with figure 2 , Attached figure 1 And figure 2 It is a flowchart of the sewage treatment fault diagnosis method based on the weighted extreme learning machine integration algorithm in this embodiment. The experimental simulation data comes from the University of California database (UCI), which is the daily monitoring data of a sewage treatment plant. The dimension of each sample of the entire data set is 38. There are 380 complete records of all attribute values. The monitored water bodies are in total. There are 13 states, each state is replaced by a number. In order to simplify the complexity of classification, we divide the samples into 4 categories according to the nature of the sample categories, as shown in Table 1. In Table 1, category 1 is a normal condition, category 2 is a normal condition with performance exceeding the average value, category 3 is a normal condition with low water inflow, and category 4 is a secondary...
PUM
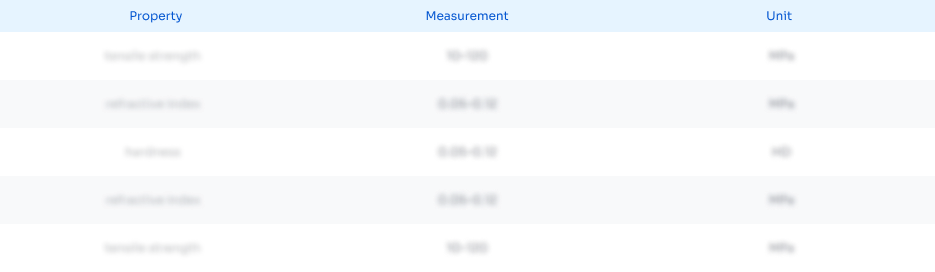
Abstract
Description
Claims
Application Information
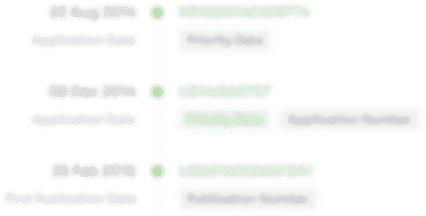
- R&D Engineer
- R&D Manager
- IP Professional
- Industry Leading Data Capabilities
- Powerful AI technology
- Patent DNA Extraction
Browse by: Latest US Patents, China's latest patents, Technical Efficacy Thesaurus, Application Domain, Technology Topic.
© 2024 PatSnap. All rights reserved.Legal|Privacy policy|Modern Slavery Act Transparency Statement|Sitemap