Deep learning-based ultrasound contrast tumor automatic identification and detection method
A contrast-enhanced ultrasound and automatic recognition technology, applied in the field of image recognition, can solve the problems of low segmentation accuracy, inability to achieve high accuracy in classifier recognition, and inability to achieve prediction, and achieve the effect of good segmentation accuracy.
- Summary
- Abstract
- Description
- Claims
- Application Information
AI Technical Summary
Problems solved by technology
Method used
Image
Examples
Embodiment 1
[0029] The deep learning-based method for automatic identification and detection of tumors in contrast-enhanced ultrasound provided in this embodiment includes the following steps:
[0030] Acquiring characteristic video data of the target area; the characteristic video is a video that is stable for a period of time after the microbubbles are injected into the tissue area completely filled with the microbubbles;
[0031] Perform data preprocessing on the feature video data:
[0032] Preprocessing: The original video is video data with a frame rate of 100 frames per second and a duration of 200 seconds. A video has a size of 2GB. Direct processing of such a large amount of data will result in too long training and testing time. The data of 1 key frame per second injected into the filling tissue for 10 seconds is used as the video data obtained by preliminary preprocessing. Among them, it is necessary to ensure that the frames intercepted between different samples have the same...
Embodiment 2
[0044] The deep learning-based automatic recognition and detection method for tumor contrast-enhanced ultrasound provided in this embodiment uses a 3D convolutional neural network to effectively extract the time-space effective blood flow characteristics within and between frames of contrast-enhanced ultrasound video data, and based on the tumor The difference between tissue and normal tissue can realize the detection of tumor tissue. This method takes into account both the spatial information around the target area and the time-varying information, which improves the segmentation accuracy; takes into account the surrounding spatial information and continuous time information; effectively integrates the nano-microbubbles in the tissue (or tumor) Flow process information is better used for tumor region segmentation. To achieve a higher segmentation accuracy, the specific steps are as follows:
[0045] Data preprocessing: intercept the video, and intercept the feature video dat...
PUM
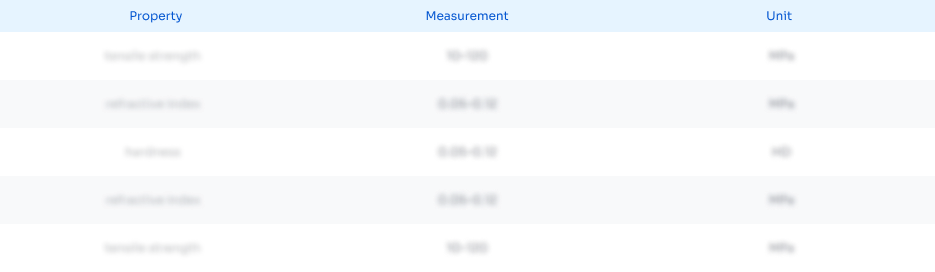
Abstract
Description
Claims
Application Information
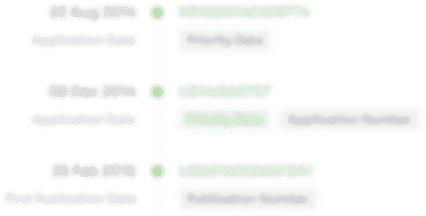
- R&D Engineer
- R&D Manager
- IP Professional
- Industry Leading Data Capabilities
- Powerful AI technology
- Patent DNA Extraction
Browse by: Latest US Patents, China's latest patents, Technical Efficacy Thesaurus, Application Domain, Technology Topic, Popular Technical Reports.
© 2024 PatSnap. All rights reserved.Legal|Privacy policy|Modern Slavery Act Transparency Statement|Sitemap|About US| Contact US: help@patsnap.com