SVR antifriction bearing performance degradation prediction method based on krill-herd algorithm
A technology of rolling bearing and prediction method, which is used in mechanical bearing testing, mechanical component testing, and machine/structural component testing. , the number of iterations is reduced, the effect of good clustering effect
- Summary
- Abstract
- Description
- Claims
- Application Information
AI Technical Summary
Problems solved by technology
Method used
Image
Examples
specific Embodiment approach 1
[0036] Specific implementation mode one: as figure 1 As shown, the SVR rolling bearing performance degradation prediction method based on the krill swarm algorithm of the present embodiment, the method includes the following steps:
[0037]Step 1: Based on CEEMD (Complete Overall Empirical Mode Decomposition: Similar to EEMD, the form of adding positive and negative pairs of Gaussian white noise has a good effect on eliminating the residual auxiliary noise in the reconstructed signal, thereby reducing the reconstruction error. Improve calculation speed) and feature extraction of wavelet packet threshold noise reduction;
[0038] Analyze the time domain, frequency domain, and time-frequency domain characteristic indicators, reflect the fault diagnosis ability of rolling bearings, and propose a new theoretical algorithm combining CEEMD with wavelet packet semi-soft threshold;
[0039] Step 2: Rolling bearing dimensionality reduction based on C-LLE (establish fuzzy C clustering ...
specific Embodiment approach 2
[0043] Specific implementation mode 2: This implementation mode is a further description of specific implementation mode 1;
[0044] Step 1 (firstly, analyze the vibration signal of the rolling bearing. When the noise signal in the rolling bearing is large enough to cover the useful information, directly using the wavelet packet threshold for noise reduction will remove the noise signal while also removing the useful signal submerged in the noise. The use of CEEMD to directly discard the high-frequency components for denoising will cause the loss of high-frequency effective signals, so the specific steps of using the method of combining CEEMD and wavelet packets) are as follows:
[0045] Step 11: Set the sampling time and frequency for the acceleration sensor installed on the rolling bearing seat, then determine the number of acceleration sensor channels, and collect the vibration signals of the rolling bearing in different damage states, and then preprocess the obtained fault ...
specific Embodiment approach 3
[0048] Specific implementation mode three: this implementation mode is a further description of specific implementation mode one;
[0049] Step 2 (Because a single characteristic parameter cannot well reflect the changes in the normal operation of the rolling bearing, and multi-characteristic parameters often have the problems of irrelevance and information redundancy, the method of feature dimensionality reduction is used to reduce the Space matrix is used for dimensionality reduction, and the rolling bearing feature dimensionality reduction method based on C-LLE is used. During the entire monitoring process of rolling bearings, not only can different damage degrees of bearings be distinguished, but also the degradation trend of rolling bearings can be predicted and classified. The normal vibration signal of rolling bearings and the final failure fault signal as training data to establish a fuzzy C clustering model. First, fault feature extraction: extract the time domain, f...
PUM
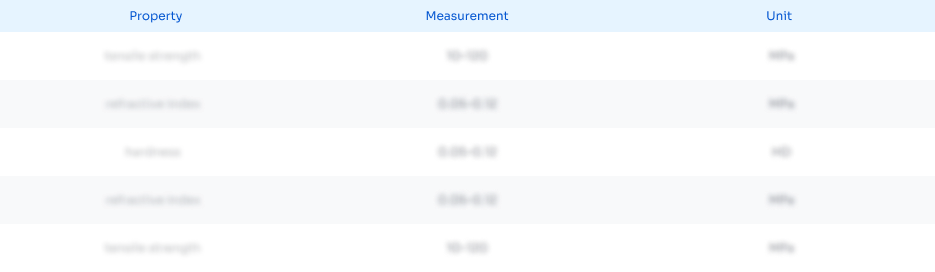
Abstract
Description
Claims
Application Information
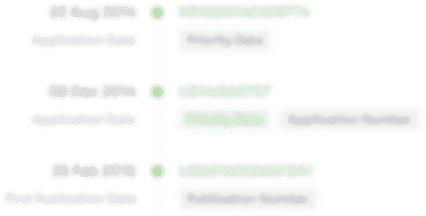
- Generate Ideas
- Intellectual Property
- Life Sciences
- Materials
- Tech Scout
- Unparalleled Data Quality
- Higher Quality Content
- 60% Fewer Hallucinations
Browse by: Latest US Patents, China's latest patents, Technical Efficacy Thesaurus, Application Domain, Technology Topic, Popular Technical Reports.
© 2025 PatSnap. All rights reserved.Legal|Privacy policy|Modern Slavery Act Transparency Statement|Sitemap|About US| Contact US: help@patsnap.com