MLE-PSO indoor positioning method based on RSSI (Received Signal Strength Indication)
An indoor positioning and indoor environment technology, which is applied to services, measuring devices, instruments, etc. based on location information, can solve the problems of unguaranteed positioning accuracy and large positioning errors, and achieves reduction of data calculation amount, improvement of efficiency, and accurate location. Effect
- Summary
- Abstract
- Description
- Claims
- Application Information
AI Technical Summary
Problems solved by technology
Method used
Image
Examples
Embodiment Construction
[0039] The present invention will be described in detail below with reference to the accompanying drawings and examples.
[0040] The principle of the MLE-PSO indoor positioning algorithm based on RSSI is: according to the characteristics of wireless sensor signal propagation and attenuation indoors, establish a mathematical model of the received signal strength (RSSI) connected to the receiving terminal and the distance between the base station and the receiving terminal, and use this mathematical model to calculate the distance. calculation. Use the maximum likelihood estimation to obtain the initialization and iteration limit range of the subsequent particle swarm optimization algorithm positioning process, use the particle swarm optimization algorithm to update the position and speed of the particle swarm within the range, and judge the position of each particle. If the particle position exceeds To limit the range is to replace the original position, and update the particl...
PUM
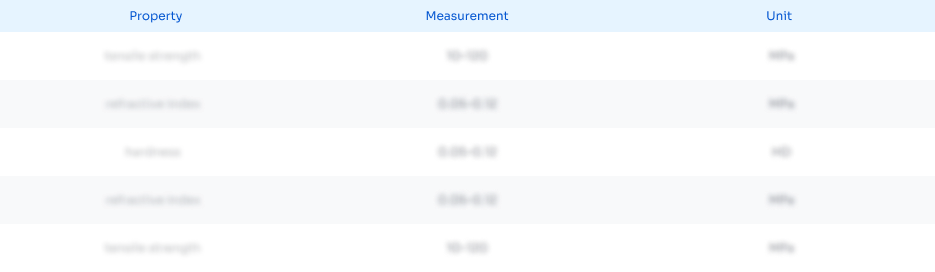
Abstract
Description
Claims
Application Information
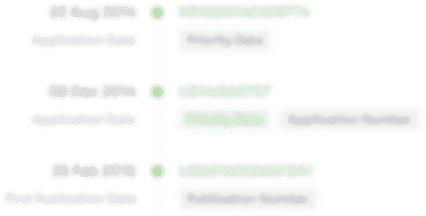
- Generate Ideas
- Intellectual Property
- Life Sciences
- Materials
- Tech Scout
- Unparalleled Data Quality
- Higher Quality Content
- 60% Fewer Hallucinations
Browse by: Latest US Patents, China's latest patents, Technical Efficacy Thesaurus, Application Domain, Technology Topic, Popular Technical Reports.
© 2025 PatSnap. All rights reserved.Legal|Privacy policy|Modern Slavery Act Transparency Statement|Sitemap|About US| Contact US: help@patsnap.com