Radar-simulation-image-based human body motion classification method of a convolution neural network
A convolutional neural network and human motion technology, applied in the field of radar target classification and deep learning, can solve problems such as partial occlusion, individual differences in human body, and multi-person recognition objects
- Summary
- Abstract
- Description
- Claims
- Application Information
AI Technical Summary
Problems solved by technology
Method used
Image
Examples
Embodiment Construction
[0019] In order to make the technical solution of the present invention clearer, the specific implementation manners of the present invention will be further described below. The present invention is concretely realized according to the following steps:
[0020] 1. Radar time-frequency image dataset construction
[0021] (1) Radar image simulation based on MOCAP dataset
[0022] The motion capture (MOCAP) data set was established by the Graphics Lab of CMU, using the Vicon motion capture system to capture real motion data. The system consists of 12 MX-40 infrared cameras, each with a frame rate of 120Hz, and can record subjects The 41 marker points on the subject's body can be used to obtain the movement trajectory of the subject's bones by integrating the images recorded by different cameras. The data set contains 2605 sets of experimental data. During this experiment, seven common actions were selected to generate radar images. These seven actions are: running, walking, ju...
PUM
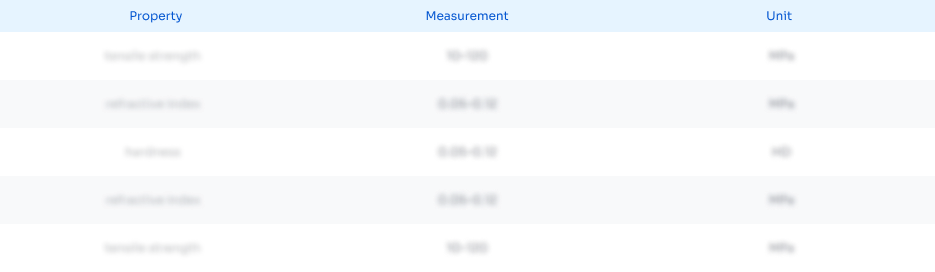
Abstract
Description
Claims
Application Information
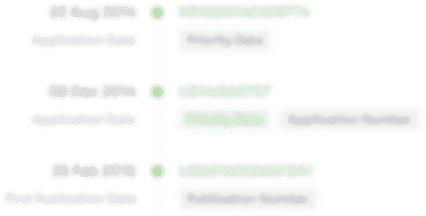
- R&D
- Intellectual Property
- Life Sciences
- Materials
- Tech Scout
- Unparalleled Data Quality
- Higher Quality Content
- 60% Fewer Hallucinations
Browse by: Latest US Patents, China's latest patents, Technical Efficacy Thesaurus, Application Domain, Technology Topic, Popular Technical Reports.
© 2025 PatSnap. All rights reserved.Legal|Privacy policy|Modern Slavery Act Transparency Statement|Sitemap|About US| Contact US: help@patsnap.com