Gamma-ray energy spectrum analysis method based on approximation coefficient and deep learning
An approximate coefficient and deep learning technology, applied in the field of gamma energy spectrum analysis, can solve problems such as difficulty in extracting effective information, reduce data dimension, low learning ability and predictive ability, and overcome the limitation of energy resolution and natural nature The interference of bottom radiation, the effect of reducing noise interference and signal dimension, and fast recognition speed
- Summary
- Abstract
- Description
- Claims
- Application Information
AI Technical Summary
Problems solved by technology
Method used
Image
Examples
Embodiment Construction
[0033] The following is based on Figures 1 to 9 The specific embodiment of the present invention is further described, and the following examples are explanations of the present invention and the present invention is not limited to the following examples.
[0034] see figure 1 , a gamma spectrum analysis method based on approximate coefficients and deep learning, comprising the following steps:
[0035] Step 1: Use the Monte Carlo method to model the gamma detector and simulate the energy spectrum of the nuclide of interest to obtain the simulated energy spectrum.
[0036] Specifically, the Monte Carlo program is used to model the corresponding gamma detector and simulate the energy spectrum of the nuclide of interest, which is equivalent to virtualizing a detector, and then placing some radioactive sources in this virtual environment to obtain the energy spectrum . Monte Carlo method, also called random sampling method or statistical experiment method, belongs to a branch...
PUM
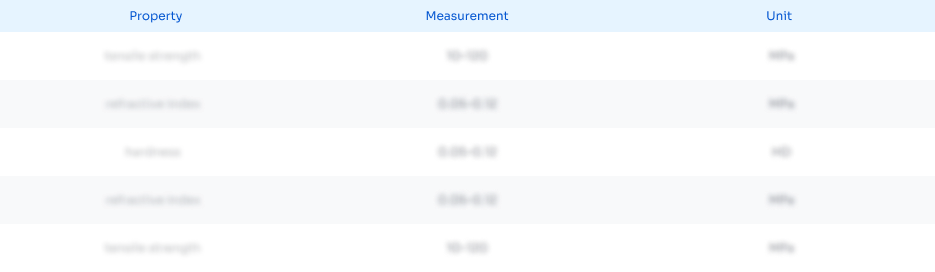
Abstract
Description
Claims
Application Information
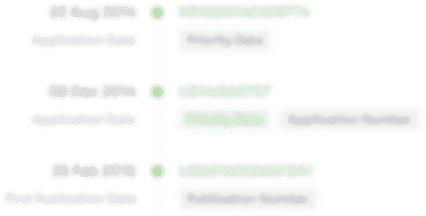
- R&D Engineer
- R&D Manager
- IP Professional
- Industry Leading Data Capabilities
- Powerful AI technology
- Patent DNA Extraction
Browse by: Latest US Patents, China's latest patents, Technical Efficacy Thesaurus, Application Domain, Technology Topic.
© 2024 PatSnap. All rights reserved.Legal|Privacy policy|Modern Slavery Act Transparency Statement|Sitemap