Tire X-ray defect/flaw detection method based on deep convolutional neural network
A convolutional neural network and deep convolution technology, applied in the field of tire X-ray defect detection based on deep convolutional neural network, can solve the problems of strong contingency, inability to accurately detect tire defects, and low reliability
- Summary
- Abstract
- Description
- Claims
- Application Information
AI Technical Summary
Problems solved by technology
Method used
Image
Examples
Embodiment Construction
[0062] The implementation of the present application will be described in detail below with reference to the accompanying drawings and examples, so as to fully understand and implement the implementation process of how the present application uses technical means to solve technical problems and achieve technical effects.
[0063] A tire X-ray defect detection method based on a deep convolutional neural network, the steps are as follows:
[0064] S1. Image preprocessing: see image 3 , to sharpen the original image. The original picture is not clear. After sharpening, the grain of the picture is clearer and the defect is more obvious.
[0065] S2. Data cleaning: cutting out the existing tire defect pictures, cutting out the defect part in the defect picture, as a negative sample; cutting out the normal part in the picture, as a positive sample.
[0066] S3. Design and training of convolutional neural network: see figure 1 , design four convolutional neural network models, tw...
PUM
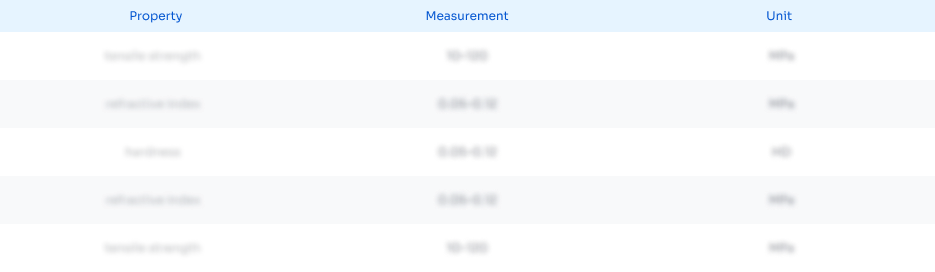
Abstract
Description
Claims
Application Information
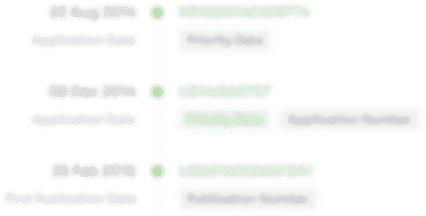
- R&D Engineer
- R&D Manager
- IP Professional
- Industry Leading Data Capabilities
- Powerful AI technology
- Patent DNA Extraction
Browse by: Latest US Patents, China's latest patents, Technical Efficacy Thesaurus, Application Domain, Technology Topic, Popular Technical Reports.
© 2024 PatSnap. All rights reserved.Legal|Privacy policy|Modern Slavery Act Transparency Statement|Sitemap|About US| Contact US: help@patsnap.com