Laser SLAM (simultaneous localization and mapping) closed loop detection method based on deep learning
A closed-loop detection and deep learning technology, applied in neural learning methods, instruments, biological neural network models, etc., can solve the problems of inability to meet high-precision laser SLAM positioning and composition requirements, low computing efficiency, and adaptability limitations. Achieve the effect of SLAM closed-loop detection, improve efficiency, and improve accuracy
- Summary
- Abstract
- Description
- Claims
- Application Information
AI Technical Summary
Problems solved by technology
Method used
Image
Examples
Embodiment Construction
[0035] Below in conjunction with accompanying drawing and specific embodiment the present invention will be described in further detail:
[0036] A laser SLAM closed-loop detection method based on deep learning, comprising the following steps:
[0037] Step 1: Construct a point cloud data sample pair dataset: collect laser point cloud data, divide the laser point cloud data into individual samples according to the time sequence of collection and a certain interval, and extract a certain number of sample pairs from the point cloud data samples. And according to the similarity of two samples in the sample pair, the sample pair is divided into a positive sample pair and a negative sample pair; construct a certain number of positive sample pairs and negative sample pairs, wherein, the positive sample pairs and negative sample pairs constitute point cloud data samples Each sample pair is composed of two samples, and the label of the sample pair is determined according to the simila...
PUM
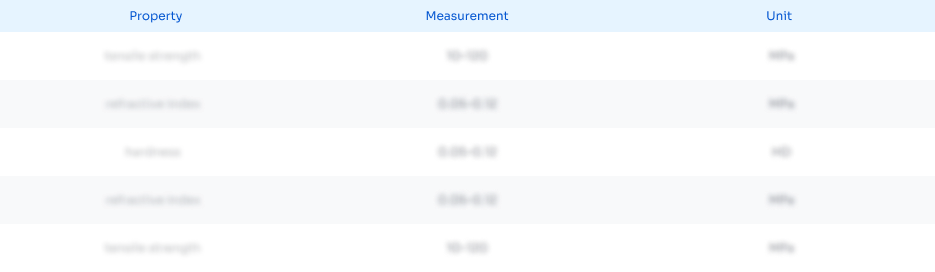
Abstract
Description
Claims
Application Information
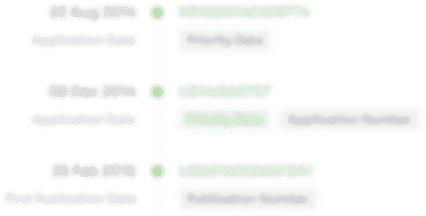
- R&D Engineer
- R&D Manager
- IP Professional
- Industry Leading Data Capabilities
- Powerful AI technology
- Patent DNA Extraction
Browse by: Latest US Patents, China's latest patents, Technical Efficacy Thesaurus, Application Domain, Technology Topic, Popular Technical Reports.
© 2024 PatSnap. All rights reserved.Legal|Privacy policy|Modern Slavery Act Transparency Statement|Sitemap|About US| Contact US: help@patsnap.com