Alzheimer's disease classification method based on depth forest
A classification method and forest technology, applied in image analysis, image data processing, instruments, etc., can solve the problems of difficult MRI image features, low classification accuracy of Alzheimer's disease, etc., to achieve early diagnosis, good description of samples, The effect of improving the recognition rate
- Summary
- Abstract
- Description
- Claims
- Application Information
AI Technical Summary
Problems solved by technology
Method used
Image
Examples
specific Embodiment 1
[0037] A deep forest-based Alzheimer's disease classification method, comprising the following steps:
[0038] Step 1: The MRI image for detecting Alzheimer's disease is used as the input of the multi-granularity scan, the output of the multi-granularity scan is connected to the cascade forest, and the cascade forest outputs a class vector of the MRI image to complete the deep forest model Constructed, the classification result of the MRI image includes Alzheimer's disease (AD), mild cognitive impairment (MCI) and normal person (NC), and the class vector is the maximum probability value in the category of the MRI image.
[0039] Step 2: Preprocessing MRI images of several known categories, that is, performing AC-PC origin correction on the MRI images; segmenting each corrected MRI image to obtain MRI gray matter images; normalizing the MRI gray matter images to A unified MNI template, so that the size of each MRI gray matter image is unified; the standardized MRI gray matter i...
specific Embodiment 2
[0042] Step 1: The MRI image for detecting Alzheimer's disease is used as the input of the multi-granularity scan, the output of the multi-granularity scan is connected to the cascade forest, and the cascade forest outputs a class vector of the MRI image to complete the deep forest model Constructed, the category of the MRI image includes Alzheimer's disease (AD), mild cognitive impairment (MCI) and normal person (HC), and the class vector is the maximum probability value in the category of the MRI image.
[0043] Step 2: Preprocessing MRI images of several known categories, that is, performing AC-PC origin correction on the MRI images; segmenting each corrected MRI image to obtain MRI gray matter images; normalizing the MRI gray matter images to A unified MNI template, so that the size of each MRI gray matter image is unified; the standardized MRI gray matter image is smoothed and down-sampled, and the processed image is sliced to obtain a preprocessed MRI image, the MRI ima...
PUM
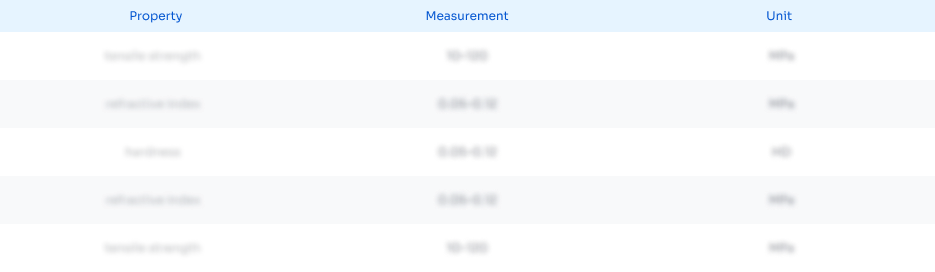
Abstract
Description
Claims
Application Information
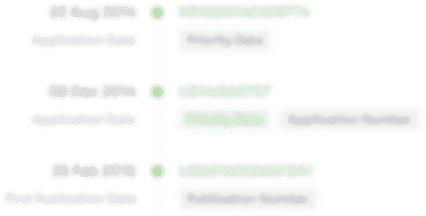
- Generate Ideas
- Intellectual Property
- Life Sciences
- Materials
- Tech Scout
- Unparalleled Data Quality
- Higher Quality Content
- 60% Fewer Hallucinations
Browse by: Latest US Patents, China's latest patents, Technical Efficacy Thesaurus, Application Domain, Technology Topic, Popular Technical Reports.
© 2025 PatSnap. All rights reserved.Legal|Privacy policy|Modern Slavery Act Transparency Statement|Sitemap|About US| Contact US: help@patsnap.com