Domain self-adaptive dimensionality reduction method through keeping maximum dependency relationship between data conversion
A technology of dependency and domain adaptation, applied in the fields of instruments, character and pattern recognition, computer parts, etc., can solve problems such as inapplicability, and achieve the effect of good applicability, intuitive physical meaning, and simple structure
- Summary
- Abstract
- Description
- Claims
- Application Information
AI Technical Summary
Problems solved by technology
Method used
Image
Examples
Embodiment Construction
[0030] The present invention mainly provides a domain adaptive dimensionality reduction learning method. The technical solution of the present invention is to map the input samples of the source domain and the target domain to the regenerating kernel Hilbert space, and then re-project to another regenerating kernel Hilbert space through the transfer matrix, and finally realize dimensionality reduction. A method for adaptive dimensionality reduction in unsupervised and semi-supervised domains. The specific principle of the present invention is introduced as follows.
[0031] make represents the source domain samples, Represents the target domain sample, then the input sample is X={X S , X T}∈R D×N , H 1 Represents the regenerated kernel Hilbert space with k as the kernel function, let φ(x)=k(·,x), and φ:X→H 1 , H 1 =span{φ(x 1 ),…, φ(x N )}. definition The transfer matrix W is expressed as
[0032] In addition to the kernel matrix
[0033]
[0034] Therefo...
PUM
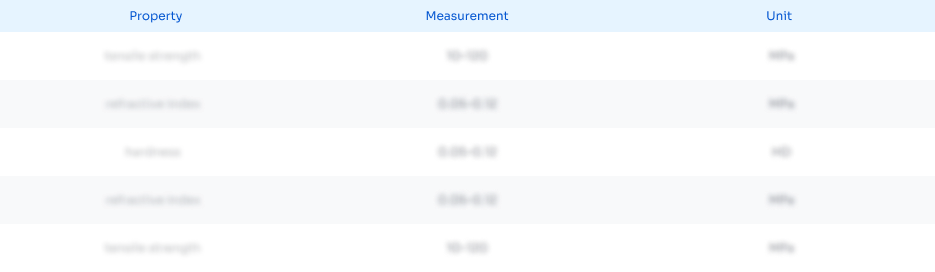
Abstract
Description
Claims
Application Information
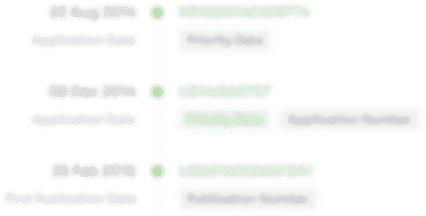
- R&D Engineer
- R&D Manager
- IP Professional
- Industry Leading Data Capabilities
- Powerful AI technology
- Patent DNA Extraction
Browse by: Latest US Patents, China's latest patents, Technical Efficacy Thesaurus, Application Domain, Technology Topic, Popular Technical Reports.
© 2024 PatSnap. All rights reserved.Legal|Privacy policy|Modern Slavery Act Transparency Statement|Sitemap|About US| Contact US: help@patsnap.com