Hyperspectral image vegetation classification method based on spatial auto-correlation information
A hyperspectral image and spatial autocorrelation technology, which is applied in the field of hyperspectral image vegetation classification based on spatial autocorrelation information, can solve the problems of neglecting hyperspectral spatial autocorrelation information and low vegetation classification performance, and achieve the removal of salt and pepper phenomenon, good The effect of high classification performance and classification accuracy
- Summary
- Abstract
- Description
- Claims
- Application Information
AI Technical Summary
Problems solved by technology
Method used
Image
Examples
Embodiment 1
[0035] Please refer to figure 1 , figure 1 is the flow chart of the algorithm.
[0036] A hyperspectral image vegetation classification method based on spatial autocorrelation information, characterized in that it comprises the following steps:
[0037] S1: Normalize the hyperspectral data set to obtain a hyperspectral image data set R with redistributed information;
[0038] where the normalization process is Among them, min refers to the minimum value of any band, and max refers to the maximum value of any band.
[0039] S2: Perform PCA dimensionality reduction on the hyperspectral image, and select the previous n-dimensional data to form a new data set K;
[0040] Wherein the PCA dimensionality reduction is K=PCA(R).
[0041] S3: Extract spatial information from all spectral information R by using domain conversion linear interpolation convolution filtering;
[0042] The spatial information extracted in step S3 is R i ds =T(R i ), i=1,2,3,...l.
[0043] S4: Extra...
PUM
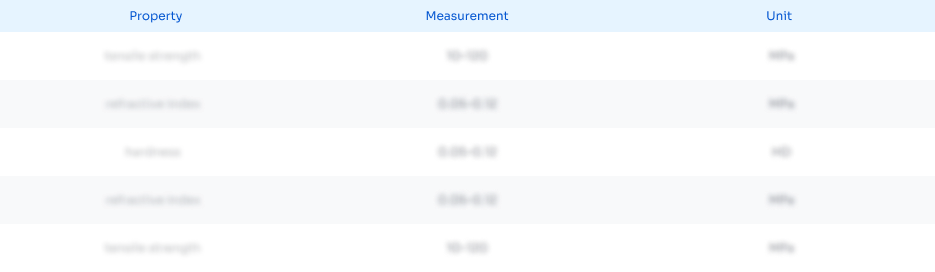
Abstract
Description
Claims
Application Information
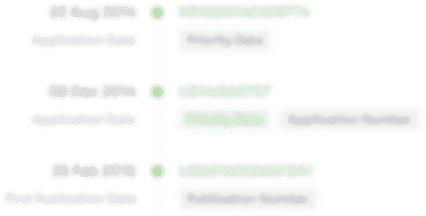
- R&D Engineer
- R&D Manager
- IP Professional
- Industry Leading Data Capabilities
- Powerful AI technology
- Patent DNA Extraction
Browse by: Latest US Patents, China's latest patents, Technical Efficacy Thesaurus, Application Domain, Technology Topic, Popular Technical Reports.
© 2024 PatSnap. All rights reserved.Legal|Privacy policy|Modern Slavery Act Transparency Statement|Sitemap|About US| Contact US: help@patsnap.com