Area-of-interest detection method based on full convolution neural network and low-rank sparse decomposition
A convolutional neural network and region-of-interest technology, applied in biological neural network models, neural architectures, instruments, etc., can solve problems such as unsatisfactory image detection results and failures, and achieve improved performance, accurate detection, and suppression of background noise. Effect
- Summary
- Abstract
- Description
- Claims
- Application Information
AI Technical Summary
Problems solved by technology
Method used
Image
Examples
Embodiment Construction
[0084] The present invention will be further described in detail below in combination with specific embodiments.
[0085] At present, the main problem of ROI detection is that the ROI cannot be accurately detected under complex backgrounds, and at the same time, the background noise cannot be well suppressed. The present invention proposes a region of interest detection method based on full convolutional neural network and low-rank sparse decomposition. The present invention can accurately detect the region of interest in complex backgrounds, and at the same time, the result map can well suppress background noise.
[0086] The present invention realizes the region of interest detection method based on background prior and foreground node through the following steps, and concrete steps are as follows:
[0087] Step 1: Input an image, extract features such as color, texture and edge, and form a feature matrix with dimension d=53.
[0088] (1) Color feature: extract the R, G, B ...
PUM
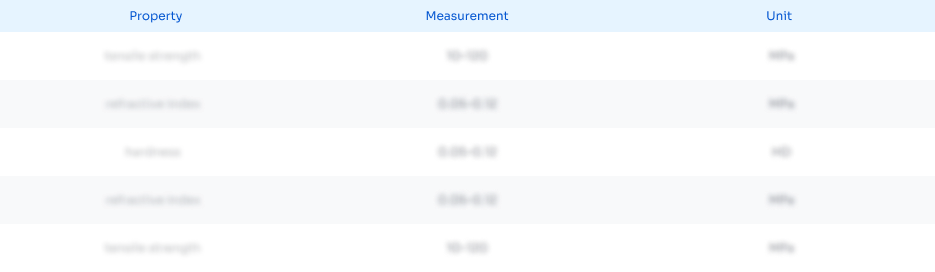
Abstract
Description
Claims
Application Information
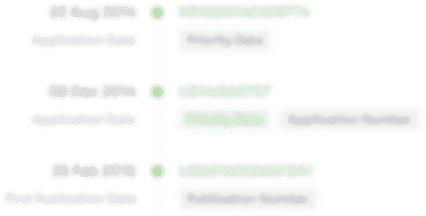
- R&D Engineer
- R&D Manager
- IP Professional
- Industry Leading Data Capabilities
- Powerful AI technology
- Patent DNA Extraction
Browse by: Latest US Patents, China's latest patents, Technical Efficacy Thesaurus, Application Domain, Technology Topic, Popular Technical Reports.
© 2024 PatSnap. All rights reserved.Legal|Privacy policy|Modern Slavery Act Transparency Statement|Sitemap|About US| Contact US: help@patsnap.com