Distribution transformer load forecasting method based on mxnet framework deep neural network
A deep neural network and load forecasting technology, applied in forecasting, instruments, relational databases, etc., can solve the problems that the time series method cannot consider load fluctuations, describe and describe distribution variables, and cannot understand distribution variables, etc., to improve data utilization Value, accurate load change trend, and the effect of ensuring safe and stable operation
- Summary
- Abstract
- Description
- Claims
- Application Information
AI Technical Summary
Problems solved by technology
Method used
Image
Examples
Embodiment Construction
[0032] The technical solution of the present invention will be further described in detail below in conjunction with the accompanying drawings.
[0033] like figure 1 Shown: the present invention comprises the following steps:
[0034]1) Obtain internal and external data of the system. The internal data of the system include GIS system data, PMS2.0, electricity collection system, online monitoring system, and marketing system data. The internal data include meteorological and macroeconomic data;
[0035] 2) Refining the acquired data to obtain load-related indicator data and historical load data;
[0036] 3) Taking the station area as the unit, through the optimal combination forecasting model and the artificial neural network algorithm, respectively fit the medium and long-term load forecasting model and the short-term load forecasting model;
[0037] 4) According to the load forecast results and index dimensions, refine the label system related to the load, and construct t...
PUM
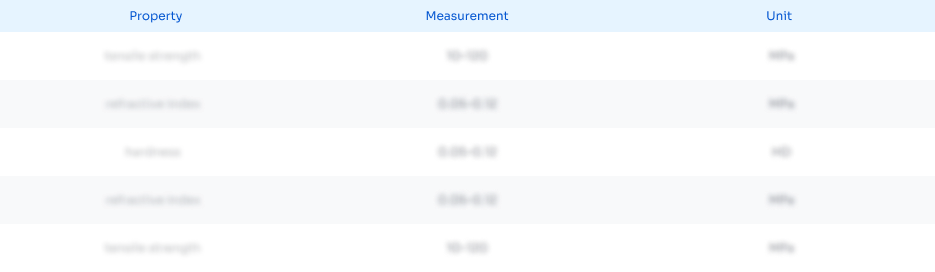
Abstract
Description
Claims
Application Information
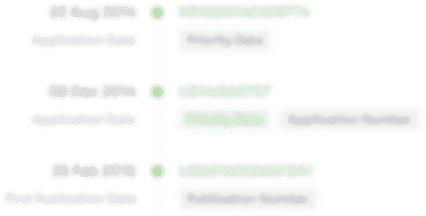
- R&D Engineer
- R&D Manager
- IP Professional
- Industry Leading Data Capabilities
- Powerful AI technology
- Patent DNA Extraction
Browse by: Latest US Patents, China's latest patents, Technical Efficacy Thesaurus, Application Domain, Technology Topic, Popular Technical Reports.
© 2024 PatSnap. All rights reserved.Legal|Privacy policy|Modern Slavery Act Transparency Statement|Sitemap|About US| Contact US: help@patsnap.com